Probability First: Understanding Quantum Computing with James Whitfield
Download MP3Sebastian Hassinger 0:01
The new quantum era, a podcast by Sebastian Hassinger and Kevin Rowney.
Kevin Rowney 0:31
Welcome back to the new quantum era. Today we've got a really interesting interview with James Whitfield, who's a professor over at Dartmouth.
Sebastian Hassinger 0:39
Yeah, Kevin, James and I actually work together at Amazon Web Services in the quantum team. And I thought it'd be really great to have him on the podcast, I find all of my interactions with James, delightful and also really, really interesting and informative. He has more of a quantum chemistry background. And he brings that sort of sensibility to his work in quantum information science.
Kevin Rowney 1:03
Great fine, Sebastian. So I guess just for for a prequel for our audience. In this interview, we'll be covering it really three main topics of one is, I mean, I thought it was really great, good, fresh, new detail on the specific areas of, of chemistry of quantum chemistry, where you might be able to sort of see adequate progress in quantum computation and its merit towards, towards cracking key problems. And then in that in that domain, so fascinating detail, I thought second topic that James got into in some degree, some detail was, you know, an extended dialogue on the intuitive nature, right, of how to perceive entanglement within quantum states, and how those manifest in quantum algorithms so really good material for people that are trying to get on top of that challenging concept. And then the third area really exciting for me was to see it to hear James' perspectives on how to better enhance pedagogy in quantum computers and quantum computation Information Science, both in the K through 12 range and at the grad level. So, yeah, three, three rich and fun topics. Good material today.
Sebastian Hassinger 2:11
Yeah, excellent. All right, here we go.
Hey, everybody, joined here today on the podcast by James Whitfield, a colleague of mine, actually a Amazon visiting academic, as well as associate professor of physics at Dartmouth University. Thanks so much for being with us, James.
James Whitfield 3:06
Yeah, I'm happy to be here. Yeah. from Dartmouth College. Yeah. It's tough. There's like a really interesting history about why it's college, not University. There was also Dartmouth University that was parallel with Dartmouth College and went up to Supreme Court. Are there two different institutions? That one one got shut down? Oh, one got shut down. It was a whole Supreme Court case. So Dartmouth University you want
Sebastian Hassinger 3:31
me to do it over?
James Whitfield 3:31
I mean, I can do it. It's a nice piece. Okay. All right. Leave it in. Right.
Sebastian Hassinger 3:38
Cool. Anyways, podcast very J.
James Whitfield 3:41
Yeah. That's, that's why anyone cares at Dartmouth? I think it should be university because they have everything for university. But right.
Sebastian Hassinger 3:48
It's very well rounded the the offerings academically, I mean, there's solid science and humanities.
James Whitfield 3:54
Yeah, sorry. I had one of the earliest courses in quantum information science, so called Lorenza Viola has been teaching this a long time.
Sebastian Hassinger 4:03
Wow. Is that where you got into QIS? Or did you had your
James Whitfield 4:08
information for a long time? So I guess a little bit about me, I yeah, I did my undergraduate at Morehouse College. And before that, I just really liked doing math. It was a nice way to kind of calm down. And so I would, you know, be frustrated. Whatever and you sit there do some integrals with you. That's great. It's
Kevin Rowney 4:25
great therapy. Yeah.
Sebastian Hassinger 4:31
Read
James Whitfield 4:33
two weeks there. That's right. Yeah. So I did math like that. And then I ended up, started off I was in pre med and then I didn't want to be a doctor at some point. But I had a bunch of classes in chemistry. And then I had a bunch of classes in math that I graduated what, what would I do next? And I went into quantum chemistry was really nice intersection of the two. I think my senior year. One of my professors choice story, taught this class or I'm on physical chemistry. And this is borderline if you change the words around you say Physical Chemistry, Chemical physics, physics of chemistry, chemistry, physics, and it's almost like, they mean nothing different. And so I took this course, and it was so much linear algebra. So from quantum chemistry, from doing math in chemistry as a major to just doing more math in chemistry, to do more math, of physics of chemistry, then you're just doing physics. And that's how I ended up in the physics department, you know, step by step by step very moving more over to physics, physics has that much better reception for quantum information science, chemistry, starting to adopt it more now. But when I went up for job searches, I was much searched in both departments. And I ended up finding a position in with what I was doing a bit of in quantum chemistry, in quantum computing, and all associated different types of projects, from quantum walks to thinking about measurements, all sorts of things inside of the realm of quantum computing, and quantum chemistry. And so doing some quantum chemistry projects around basis set designs, I have some fantastic graduate students thinking about this. So we've been playing around with the mathematics thinking about how the tooling goes thinking about what a second quantization, how do we transform things from being descriptions of quantum chemistry to descriptions that are of qubits? How do we take that and start thinking about how that applies more broadly, to locality of systems? How does that apply to Tensor Networks? How does it apply to different aspects of mathematical Physics, Chemistry, Chemical mathematics, and it's just a nice fun to play?
Sebastian Hassinger 6:36
What's the simplest way to explain what quantum chemistry is, as opposed to you're not talking about quantum computation applied to chemistry, quantum chemistry is sort of a subset of chemistry itself? Right?
James Whitfield 6:47
That's a great question. So I would say, the way someone explained it to me a long time ago, at my first internship, he said that the goal of computational chemistry is to put all the wet chemists out of business, just be able to see the type of the algorithm and write back an answer. And with machine learning is becoming more and more of a reality. But nonetheless, the praying is that that was the goal of computational chemistry, you do not have to mix the chemicals to find out what happens out of reaction, to figure out what geometry it is you don't need to shine a laser, you don't have to do spectroscopy, you don't have to plug in a mortar device at all, you have some computational way of justifying what you've seen, physically, or guessing what you should see physically when you go to experiments. And so it's way of complementing experiments. With theory. That's where computational quantum chemistry comes in.
Kevin Rowney 7:38
And that very remarkably, that's, that's a great, I think, lead it to this sort of interesting area that motivated us to invite you onto the podcast. And again, really thank you for for making the time. But you know, there's so many people in the quantum computing, you know, ecosystem that point at molecular geometry, physical chemistry, all that stuff, as one of the lead possible outcomes, right of quantum computing, showing, you know, huge breakthrough new results. And it just appears to be that there's actually a lot of, I don't know, maybe that poorly, well understood, poorly understood facets of those problems in quantum computing. So we were hoping to dive into that into some detail. Do you do you have any framing remarks on the you know, the merit of quantum computing in this early phases in terms of its applicability to physical chemistry?
James Whitfield 8:28
Yeah, I mean, I have a few. I think, one of the most interesting things, I think both definitely inside the news media, okay, it's not even trying to reach these guys, because it's a bit hard to really explain all the details. But even in the scientific community, there's quite often a missing piece that a lot of people skip over when they're thinking about what to do with the quantum device, these variational quantum eigen solvers. I think this is what you mentioned, geometry, optimization, all these optimization problems. All are heuristics. There's no guarantee you will get a speed up. There's some controversy on the archive now about Grover's search has no speed up, that I haven't read the article and will come at any more than to say, I don't think I buy wholesale. Okay. I don't know what it says. But I just, you know, the point being is that on these problems that are NP hard, the most you could do, is Grover speed up. And so that's the best you can do. Okay, this one question implementation, which what I think of paper gets that, but optimization is not where quantum computing expected to make huge breakthroughs. And so what I think the main missing disconnected side of the community about how computational chemistry can be used with respect to quantum quantum computational science, so computer science thinking about what can be done. per computer science, optimization of quantum wave functions is extremely hard. This is the quantum Merlin author class. However, this means that you shouldn't expect quantum computers to do any You better. I mean, maybe better, maybe not depending on heuristics, but there's no promise there. And so all these optimization problems don't have any promise other than NP, rather than square root speed up when something like using Grover's search
Kevin Rowney 10:12
Per Grover? Yes, makes perfect sense. It's an excellent kickoff, and it got really a fascinating topic for me. Yeah.
James Whitfield 10:20
On the flip side, there is this notion of time evolution. When you do time evolution, this is very different than thinking about optimizing. And so this is where a lot of I think a lot of distinctions lost, even inside the inside the elite community with people who really building quantum algorithms. So not always thinking about how dynamics can be used, and how that can be utilized to do something. And so thinking about problems that are the dynamical nature, rather than optimization in nature, are really two different complexity classes. From a computational computer science point of view.
Kevin Rowney 10:53
That's really, very, I think of apt remark. And our audience has got a diverse array of different levels of depth of mathematics. Could you maybe just for some of the people that are more of the tech end and not math hobbyists, you underline and more deeply elucidate that that distinction.
James Whitfield 11:12
Yeah, no, of course. So if you think of optimization, you want to find the best way of doing something. And this is saying that you have many different settings, you have to go to all the different settings and find what is the what is the best, you know, what is the optimal for whatever you're trying to optimize, could be anything. This is very generic set of problems. But these problems generically hard. Generically, just, there's a complexity class of difficult problems. And you can classify all problems that are extremely difficult to certain set of problems that you can answer quickly, that you can check the answer quickly. And this is true, whether new quantum or the classical setting, but this is just from problems, we have to try every instance, if you get the right instance, you can check it easily. But getting the instance you have to go over all settings. And this is one class of problems is called optimization. And this is very broad class. On the flip side, when I say time evolution, what I mean is that you give it some initial state, and you just want to propagate it forward in time. So you can take one of the really fantastic stories is thinking about modeling the solar system. So you know where the planets are, you have some additional set of conditions. And the Think about where we'll be a month from now you have some data, and you see how it goes forward in time. Depending on the exact problem, you can propagate forward in time efficiently or not. The for the solar system, if you don't include the interaction between the planets, you can do forward in time as far as you want. However, if you however, if you include the interaction between the planets, it becomes chaotic, and you don't really, really propagate so far into the future.
Sebastian Hassinger 12:45
And is the analogy there that you can model the relationships through entanglement in a gate based quantum computer. So that's,
James Whitfield 12:53
that's a that's close, I think I would phrase it slightly differently. If we think about what happens inside of quantum time evolution, is that your entanglement is growing linearly in time, this is whether us gate base or any other sort of situation where you're doing some sort of entangling gate, it that's part of the evolution, which generically speaking, it's part of the evolution operators, you have to be at the very least, something very clever to make it not universal. And so if you have a universal set of gates, when you propagate in time, then you just expand out. And you'll see what happens later in time. However, the entanglement of that system grows with the length of time you're going such that classical simulations, for example, tension networks will fail, just because the entanglements growing in time, the number of variables you have to keep track of is growing in time. And so it's comparing to
Sebastian Hassinger 13:44
the computational. Right, the computational resources required are growing exponentially.
James Whitfield 13:49
Exactly. Well, yeah. For the algorithms we have for the classical algorithms. Exactly.
Kevin Rowney 13:54
Interesting. So look, you know, just as a hobbyist of studying this, I'm no accomplished scholar. But I mean, just as a hobbyist, it's clear. I mean, there's been almost 100 year quest, right, in terms of applying, you know, the axioms of quantum mechanics to the area of chemistry to find these new algorithms. It's a very well developed field with still lots of unsolved problems, but still lots of progress. And so, I mean, again, it feels like many people the QC community, that point at this this area as something which feels like, you know, quickly within grasp, what are the very first QC problems that will be cracked, you know, molecular geometry, but you see news showing, it's not at least some doubt about that. So do I read your club correctly?
James Whitfield 14:41
I think I think I think I'm kind of not getting across exactly what I want to say. So it's not so much that I think quantum computing for geometry optimization geometry, optimization is only one problem that people discuss there's many problems that are discussed Yes. And in most of the literature's around electronic structure you optimizing the electronic wave function. This is where you're not doing geometry optimization, geometry optimization, when we're thinking about the nuclei are, of course, people do this as well. But normally, when you do geometry optimization, you can apply classical mechanics. And this is where you get these really nice molecular mechanics, experiments, molecular dynamics. Um, these are almost all classically dumb electronic structures that dictate what are the binding energies? What are the rotational constants, what are the things that are holding the things together, then you model a springs later. But to actually get what the strength of that spring should be, you need to know what the electrons are doing. And that's where electronic structure comes in. And this is where a lot of the effort inside of quantum computing has gone in. And that's where my work has been. For almost, since 2006. I've been thinking about electronic structure and quantum computers. But these electrons are dictating where the nuclei are, what the force is nuclear feeling, which way they're going to move. Next, you know, what the forces on them arm? What the rotational moment to moment of inertia, the all these sorts of quantities are coming from electronic structure. And so this is why this is a huge important aspect of thinking about how do we get to molecular dynamics, you have these these very well characterized potential energy surfaces from the electrons moving around, then that dictates where the nuclei will be.
Kevin Rowney 16:17
So and you seem to be touching on what's called commonly the the Born Oppenheimer optimization. Yes, that you could perhaps start with a good guesstimate of the position. Did I say that? Please? Forgive me if I if I said that wrong. Yeah, the Born Oppenheimer approximation, right? If not optimization, yes. And it places the nuclei. And then you the the larger problem is, well, it's the configuration of the electrons, which is a much harder problem. Do I have that right?
James Whitfield 16:46
I would say an easier way to say that is that it's also called the clamp nuclei approximation, aha,
Kevin Rowney 16:51
much better, much easier to say. Yeah. And
James Whitfield 16:53
also, I think, more visual, because you can see the nuclei cramped, clamped into one spot. And then you think about how the electrons are moving around them, and you clamped in a different spot. And so electrons are moving around them. And so you clamp the nuclei, and then you let the electrons move.
Kevin Rowney 17:07
So for that, that ladder problem that the much more difficult one of the the shape of the electrons, as they cluster around these nuclei, that comes from a solution can be found if you've got deep inside on shortages, equation for, you know, quantum correlations between these electrons. But that's a hard problem to solve. Analytically, it's really impossible analytically and very difficult, very challenging numerically. Yes.
James Whitfield 17:34
So there's two ways to think of the Schrodinger equation. If you go back to wherever, may may or may not have heard of it. But in soldiers equation, you can think of it as linear equation, telling you about eigenvalues and eigenvectors, we can think of it as differential equation telling you about time evolution. And these are not at all the same approach to thinking about the problem. When you think about finding the lowest energy state, you're solving the eigenvalue problem. The other case, I'm talking about dynamics using the Schrodinger equation as a way to propagate in time the differential equation version. If you do the differential equation version, this is what quantum computers are promised to do. On the complexity class, VQE VQE, this is just doing time evolution of the quantum device that is followed Schrodinger equation as a differential equation. And that's what quantum computers can do. Well, that is thinking about spectroscopy about excited states about what happened, when you hit it with the photon, how the system evolved from there. These are problems in quantum chemistry that people have not been approaching for hundreds of years, or for 100 years, rather, because what they've been doing is ground states. Yeah, there's Yeah, sort of heuristics for solving for ground states for figuring out what geometry it is, you know, what is the configuration of that state? You know, what is the spin of that state all sorts of properties in the ground state and an equilibrium we have fantastic tools for understanding molecules that equilibrium states in their electronic structure, but we start talking about the excited states, what is the ordering of the spin states sort of questions that nature become much more difficult? These are questions that you can answer with the quantum computer by doing dynamics, God, and this is where you start to see dynamics coming in, when you think about this, just to kind of maybe wrap a little bow around it that might help us, you guys or your listeners, is that we think about dynamics. And you think about what is what is spectroscopy, quantum chemistry context, you're doing the Fourier transform of something. You've done the time evolution that you fully transform that time evolution. And if you think about the heart of Shor's factoring algorithm, that's also a quantum Fourier transform. So you can see the time evolution is the same game. You're doing a time evolution, you're doing a Fourier transform over and that's telling you about some of the spectra of something that's interesting, but that's a dynamical quantity. You're getting out it's not about optimizing initial state to take an initial state characterizing it in time. What happened
Sebastian Hassinger 19:51
interesting, and in terms of applications, James, like, what types of things would you be able to do if you could model those those time intervals? because that was dynamic,
James Whitfield 20:01
that's really interesting. It's really interesting. I, you know, I would say that as quantum chemists, it's not been looked at as much. They think in many ways, the fact that, you know, there's not as many ready to go applications for what you can do if you have time dependent. Quantum control, is because when you have a hammer, everything looks like a nail. Yes, yeah. There's a lot of experience in solving ground state problems, you know, from DFT, and different techniques of DFT. And how to optimize the code, how to use, you know, GPUs, you know, to use screensavers, on and on and on, and all these different methods classically, that have branched out all around finding this ground state and better heuristics for it. So the heuristics for ground state are actually really good, which makes it seem like not a great place to try and get a quantum computer to do something. Because the heuristics are really good already. Why should we expect that we'll be able to get a better heuristic, or variational problems, we already have great heuristic, classically, we're looking at very niche, very dark corners to try to find, you know, Jim, when there's a whole big array of light that no one really knows how to tackle, right? And so it's not well,
Sebastian Hassinger 21:08
and we can marshal, like millions of CPUs and, you know, have incredible accuracy and resiliency and fit and fidelity in our computations classically. And on the quantum side. It's kind of it's, it's in a very nascent state, let's say, yeah, so yeah, it does seem like a the wrong place to start looking. So. So what you're saying is that, essentially, its familiarity that's driving the early explorations in using quantum computers for quantum chemistry. And your gut feeling is that that it's, you know, it's in the areas we haven't tried doing with computers yet, because there's just too hard classically, that might yield the first result. That's interesting.
James Whitfield 21:50
I had a few colleagues. When I was in grad school, they gave talks about doing chemical reaction dynamics, and they just, you know, doing h2 plus h, that's like, where they're at, you know, huge problems, and they have to do this surface. So you know, where they're at. Now, it's to say, it's not many quantum chemists working on, it's not a huge community. I mean, there are some, some codes that do time dependent tddft, things like this, that are that are there. So it's not saying it doesn't exist at all. So I don't want to, you know, putting my colleagues out there, you know, out, but there's a lot more space for more to be done. And it's not as commonplace doesn't work as well, you know, groundside DFT, works fantastic. tddft, they're, you know, thinking about what ways to make better approximations, just not as well-trodden. This is a lot of things around what excited states, what happens when you hit somebody with the laser. If you think about some applications of Boson sampling problem, this was to molecular spectroscopy, rovibrational spectra. So this also is another place that you can see spectroscopy coming to the forefront of what's happening, thinking about time dependent, quantum quantum mechanics,
Kevin Rowney 22:58
this is really a very fastly conversation for us, because for a long time, and many of these interviews and conversations, again, there's this is this nuance of the difference of the types of domains where you could expect to see progress, it's really not been underlined. So this clear separation between these subdomains, people just
Sebastian Hassinger 23:15
say chemistry.
Kevin Rowney 23:18
But also it's really, it's so illuminated here, you sort of so directly pointed how there's this long history of this focus on the ground state optimization problem, not solved. But boy, there's been a lot of progress and with the rise of ml even more, but yet these other dynamics areas, I mean, who knows there could be a gigantic new outbreak of like huge breakthroughs right in, in chemistry from this very domain. It's just it's almost unstudied at this point, because it's so hard.
James Whitfield 23:43
Exactly. I mean, it's a good way to summarize. And James is,
Sebastian Hassinger 23:50
does it require like you were you were talking about, like VQE is an approach and that's, that's in large part sort of trying to figure out useful things we can do with NISQ machines. Do you think that what you're describing in terms of time evolutions, you need fairly large numbers of fault tolerant qubits to do useful calculations? Or is that something that NISQ Or even analog devices like QuEra might be able to be helpful with?
James Whitfield 24:18
I think there's some pretty easy things that will convince me You know, I mean, this is Simone told me once to give away my Legos. And you see, one point I didn't like, Give me all my best ideas, but like, I let's brainstorm together guys, right, like, yeah. What did you know, one of the ways that I would be convinced that quantum computers on a path to doing dynamics, interestingly, is just seeing how much entanglement entropy you've generated over time. And I think this is, I think, an easier way to think about the random circuit sampling rather than saying random circuit sampling. Okay, maybe it's hard to sample from maybe it's not, but just thinking about how much entanglement you can generate. I think one of the one of there's a number of tricks about how to get around the random circuit sampling and one of the ones that I really liked I was at a mission Luke in some of the guys over at Harvard, and I think MIT. And what they had was just a way of breaking up the problem into smaller sections, I think stitched together and you could do it on one processor. It was like real cheap hack. It was
Sebastian Hassinger 25:15
sort of like circuit cutting and circuit knitting as though you're
James Whitfield 25:18
referring to something to do with the statistical nature of the, the benchmark that they're using. And because they don't sample the tails, you can you could cheat it. So basically, some web hacking statistic, and they could beat the the random circuit sampling. Oh, I
Sebastian Hassinger 25:34
see. You're saying classically be the random circuits. Yes,
James Whitfield 25:36
yeah, one processor. Right. Well, and
Sebastian Hassinger 25:39
in fact, I believe during during our visit on that paper, we just recorded a conversation with her a couple of weeks ago. So that's, that's coming out shortly. Yeah, there's a whole bunch
James Whitfield 25:49
of papers about this. But I think a lot of the some of the statistical hacking, they're just ways of kind of attacking the way that you've measured your statistics. But I think entanglement entropy or something of that nature to actually say, how correlated Are you across the whole number of qubits that you have? You can correlate, so many qubits evolved them in time, right, there'll be some point at which I say my time dynamics from a class computer can't do that length of time. And I think that would be maybe not an easy way. I haven't really ever crossed all the T's out of the eyes, but some clever graduate student is waiting.
Sebastian Hassinger 26:24
Some researchers have have looked at like, how large a GHZ state they've been able to produce, I think on one of the IBM Systems they were. So that's, that's not that doesn't meet the
James Whitfield 26:37
bar, GHZ, is very low. So when you write down the tensor network that is very low balled dimension, so Okay, okay. It's too sparse. Yeah. It's just not hard to simulate with the tensor network.
Kevin Rowney 26:48
Oh, yeah. Yes, fun conversation at a high level. And again, for our audience that may not be as committed fans of studying the mathematics, we could we just like unwind a little bit. So this this entanglement entropy and its growth over time, I think this is a wise remark. Could you sort of dig into it a little bit more just for our audience and give a sense about what is entanglement entropy? And what does that mean for computation? That's because it's a cool topic.
James Whitfield 27:12
So entanglement entropy, I guess, for to kind of zoom away from, quote, Information Science, I think maybe they say what entanglement it is just some very easy level, my way of thinking about probability, my way thinking about quantum mechanics and my entry point to understanding all the quantum mechanics has really been through mathematics. And so when I think of how to describe quantum mechanics, somebody who's never heard of it, I always think start with probability first. So this is a writing papers about probability first, and trying to figure out the best way to kind of teach someone who knows nothing about quantum, what is quantum mechanics. But if you start with probability, think of a probability vector, and how probability vector can transform you into Markov chains and some restrictions as to what can happen, etc, etc, you can do the same thing for quantum mechanics, if you treat the states in the right form, you start asking you about how these states evolve and how they change. And this will then give you back all the formulas that you have from quantum theory quite nicely. So this is kind of how I think about what what quantum theory comes down to. And so entanglement is just stronger than classical correlation. So we think in terms of probability, if I have, you know, athletes playing a sport, you can imagine that athletes that play basketball, might also be good at playing handball, because they're, they're used to, you know, moving their hands around, and someone who does, you know, wrestling might also be good at doing jujitsu. And so you have these kinds of correlations, because, you know, there'll be good at one sport moreso, than someone who's switching completely from basketball, to a combat sport, where they've never really, you know, had to contact somebody, you know, touch the touch, right? And the flip side, somebody plays Judo doesn't practice catching balls, or whatever moving their hand eye coordination. And so these things are correlated variables. This is correlation, however you want to think about it. And there's different measures of correlation, mutual information between two variables, you know, the entropy can be used to think about, you know, what is the, what is how much missing information is there. And so all these terms are from information theory and thinking about correlations in different ways. It's a technical technicality. And so we've got a quantum variables, you have the quantum state that emits and this whole idea started with probability. First, the concepts don't change, you still think about two quantum subsystems are correlated, you know, you have the electron, the electron has a spin maybe has location in space, and these two variables might be correlated. These two degrees of freedom are entangled if those correlations go beyond what is classical correlations. But these are just another way of saying correlations. There's no real distinction between correlations in the quantum in the classical case other than the quantum ones could reach higher measures. This is Bell's inequality,
Sebastian Hassinger 29:49
or is it also isn't it right? Is it also saying there can be correlations in quantum systems that can't exist classically, you have
James Whitfield 29:57
to be very careful. There's a bunch of technical statements that are all like, Applicable here. But like the ideas. Yeah, that's exactly right. I mean, more or less. So if we don't get the technical guys to quantum philosophy, they really think about what are the implications of, you know, Bell's inequality being violated by, by that about beyond Bell's inequalities, it's not local boxes. There's a whole lot of theory behind this, though. Yeah, it's all related to correlations.
Kevin Rowney 30:25
Yes. And again, just for the sake of our audience, it just feels like the the soul of the claim for many people in terms of their justification for believing in the merit, the extra power of quantum computing is largely founded on the capacity of these systems to witness entanglement and have a richer notion of state, which is harder to express classically. So I mean, do I have that right. Am I on target on that? That's
James Whitfield 30:50
100%. Right. I think that's a at the heart of what is quantum computing? It's, I would say, more fundamental than entanglement, I would say, coherence. Yeah. So here it is really what's allowing these correlations to be much stronger. And that's really how you can build these entanglement that grows at time because you have all these coherence is that allowing things to interact differently than if they were incoherent? And if they're incoherent, then you're just back to ordinary probability theory. So what are the correlations you could generate with a Markov chain? Okay. And then you start thinking about how coherence is what are the correlations that can move beyond what I have at the Markov chain. And this is how you can think about how the quantum extension of probability starts to give you more power. So this coherence is where that ends up being useful entanglement or useful in some other notion, you know, syncing, for instance, you know, last week coherence is might be another way to characterize the environment, there's a lot of things you can use as a coherence is for that, I think, a more fundamental adjusted temperament.
Sebastian Hassinger 31:47
And then circling back to Kevin's original question. So how does that relate to increasing entropy and taking
James Whitfield 31:59
entropy is just a buzzword for correlations?
Sebastian Hassinger 32:06
And would you say coherence is like the simplest way to understand this, that the period of time that that a qubit is is controlled is, you know, is able to be managed before it sort of its state falls apart becomes noise?
James Whitfield 32:18
I wouldn't I wouldn't characterize it that way. I mean, that's that's the time.
Sebastian Hassinger 32:23
No, I'm saying yeah, I'm saying but the coherence is the time before it becomes decohere. It is under control.
James Whitfield 32:32
I wouldn't put it that way that to say that I disagree with that definition. I think it's a reasonable definition. But I think a way that I was meaningful here, it's there's not so much in terms of how long you are coherent, but rather what you have when you are coherent. And so when you are coherent, okay, it any sort, you know, even slightly coherent, or whether completely if you completely decohere it, then you have this a probability distribution. Exactly, probably distribution, there's nothing at all that you start. So if you think about the probability distribution is a vector. Okay? Imagine everybody imagined a vector inside your imaginary, you know, podcast world, space through that vector, the diagonal of a square, that all the off diagonal parts that aren't that vector are zero at the beginning. And if they're not zero, then the coherences, those are all the off diagonal elements of that matrix. And so all those off diagonal elements, that they all disappear, then you completely declared, and they start to populate, then you have some coherence, we have a little bit of coherence, you can simulate this classically, it's not that interesting, you have a lot of coherence, then it starts to allow you to correlate variables in different ways to detect things about your environment, to you know, you know, do super dense coding, whatever you want to do now using these off diagonal elements that give you more ability to correlate random variables. And if you just had a vector, now you have a matrix to think about everything the density matrix, right.
Kevin Rowney 33:57
Yes, yes. And so this this, this entanglement Entropy is a measure right to the to the extent the propagation of this entanglement coherence, the and what you're sort of saying I gather that is that you're measuring the the rate of growth of entanglement entropy over time, is at least one way to capture the richness of the other quantum state, but eath To what do I have that correct?
James Whitfield 34:22
Yeah, but it's a pretty pretty, pretty close, right? I think. Yeah, I think your summary is pretty spot on about, you know, thinking about how the time evolution causes correlations to build up or those correlations more than you could build up classically, I think is maybe a simpler way of saying it without using entanglement at all. Just keep it simple, like you said for your everyone
Sebastian Hassinger 34:48
I think entanglements is still hiding in the word court is what like,
James Whitfield 34:53
don't use it was just a correlation. Yeah, and right. I mean, who knows what correlations are anyways, right?
Kevin Rowney 35:00
So I wonder, are there any specific algorithms or facets of quantum computing where you there is this this, this idea about measuring the rate of growth of entanglement entropy gives you insight or around the specific nature of the algorithms or their their merit for quantum computation? I mean, help us frame that, that that, that that specific mode of analysis in some way that would help help us see the landscape of the more clarity, I guess.
James Whitfield 35:28
In many ways, the random circuit sampling problem was designed to do something akin to this, and I guess, you know, surgery boy, so And these guys thought about it for a long time about what to do, they still get defeated by one processor,
Kevin Rowney 35:41
man. Yeah.
James Whitfield 35:46
But you know, if you think about what, what it's hard for a classical computer to do, think about something like Tensor Networks. So you can measure when tension networks are having trouble to capture the state by what the bond dimension is, this is a way of saying correlations. Again, so technical way of measuring the correlations. And so you can just ask when your quantum computer has generated enough correlations, you can simulate it any longer tensor network,
Sebastian Hassinger 36:09
in practical terms is really just Okay, so I had two questions occurred to me one, I feel like I'm repeating myself, maybe I'm just grabbing this, but but when you talk about like the numbers of correlations with that roughly, in in chemistry, going back to that the application in chemistry, with that sort of be analogous to sort of how, whatever complex the chemical reaction, that chemical system is that you're trying to simulate?
James Whitfield 36:38
Yeah, there's so it's really, it's a mess, really, when you go from, you go from make sense to quantum chemistry, like communities are so different, that different, but it's very smart people on both sides of the fence in, in quantum chemistry, everything's anti symmetric because of this fermion thing. And there's a huge controversy about whether the anti symmetric causes them to be correlated already. Because many ways, you could say no, that's not enough to be correlated, doesn't really count for anything. But then you have a very different notion of what a product state is, if you allow it to be anti symmetric, or if you don't. And so this is a big a whole sub literature about entanglement. It's at fermionic systems, that does not line up with any literature inside of quantum chemistry. So it's just about correlation of almost like,
Sebastian Hassinger 37:29
Do you Do you feel like torn between these two worlds, because it feels like there's very different sort of, you know, intellectual framing at this point in time in those two arenas.
James Whitfield 37:42
So at the risk of giving away my my secret .
Sebastian Hassinger 37:50
I'm reading my application,
James Whitfield 37:52
all the way that I've been able to manage like at Dartmouth, so I started AWS about two years ago. But before that, I was, you know, managing a team, and it's me and some undergraduates that show up occasionally, or they come through I had some really good undergraduates, but, you know, some of the best undergraduates came to my group did fantastic things we learned together and didn't finish anything. And they just left it like, I don't feel like it was okay, bye. And that's it. And then the graduate students come, they spent five years by time they're trained, and excellent, they're ready to solve a problem separately. If you don't, it's like, oh, I can't, I can't, you know, I can't compete with like, a full team full of PhD students full of you know, you know, the top, you know, talent or whatever, to hire the best postdoc, if I get a good postdoc, they go work for these guys. The next year, my best graduate students leave the work for these guys. So I had to pick a topic that was interesting that no one's really working on. That's really my way of kind of playing the game. And so the way I looked at it is there's a large overlap inside of what's happening inside of quantum information science, what's happening inside of condensed matter, science, and what's happening inside of quantum chemistry that don't line up at all. Not that they don't line up in a bad way. But there's a lot of mismatch and ease in terms of motivation. So I think people in say, so one of the one of my, one of my first articles I wrote coming out of grad school was computational complexity and quantum chemistry. This paper was a computational complexity electronic structure. This was with Peter love, and Alan Asperu-Guzik, and this is actually started off as a term paper for Scott Aaronson's class
Sebastian Hassinger 39:23
cool. name dropped much Peter Love, Asperu-Guzik and Aaronson Oh, in one. Yeah.
James Whitfield 39:34
Scott was on my committees. I was alone.
Kevin Rowney 39:37
Nice, man.
Sebastian Hassinger 39:38
Yeah. Is good researchers, all of them.
James Whitfield 39:41
Yeah. So Scott wrote a paper about why quantum chemistry is hard. And it was a comment on on this paper about DFT and in QMA harness this Quantum Merlin-Arthur NP-Hard. Doesn't matter if you don't know the buzzwords, but this is the optimization problem. So he's saying that this optimization problem Quantum Chemistry is very difficult. It's as difficult as other difficult problems that we know of. And that was what the crux of the paper was. And Scott had written and written the news and views for nature. And I was I was, I was so caught, I was so excited to read this, you know, it's someone in the chemistry department. And it's curious, like, oh, man, there's some proof that why quantum computers can be useful in quantum chemistry. So I went down the whole rabbit hole, I sat in the class, and tried to follow as much as I could of what's gonna teach me about computer science. And then at the end, I wrote this paper about how this implicates electronic structure. And this paper was very hard to get published very hard to get published, it's very hard to get past referees at the Chemistry Journal, they're like this not chemistry shouldn't send it here, you know, this should be like this, you didn't explain this, whatever, whatever, whatever. But for me, it was also a place that no one's working. Right? It's a place that has a lot of intellectual degrees of freedom, a lot of places to work with people, a lot of places to learn from people, a lot of places to do new work that no one else is thinking about and tensor networks, almost all the Tensor Networks on lattices is fantastic work by these guys thinking about Lieb-Robinson bounds and tension networks inside of, you know, 2d and one dimensional systems. But what happens when you don't have any dimensionality. So in quantum chemistry, you pick these basis sets that are the s orbital, the p orbital, the d orbital, if you've ever seen like these, these blobs, whatever, but these things are huge relative to the size of the system. So the s orbital, the three s orbital, the two s orbital of carbon is going to be as large as the full hydrocarbon. Usually, that hydrogen orbital will also be the same size. And so there's no notion of lattice here. And so this really changes how you look at what physics you're learning how these algorithms are applied, which algorithms work which algorithms don't. And so we've been thinking a lot about basis sets in my group. But this is really one of the places that really causes a mismatch between quantum chemistry and condensed matter, and quantum information science. They think Quantum Information Science will allow us to bridge that in a very interesting way. That's really what I have my group working on, what we're trying to do before everyone realizes how cool this is
Sebastian Hassinger 42:11
well the cover is blown to at least a few 100 people here, this gives them
Kevin Rowney 42:17
a little bit of a decent moat. That protection, though, right? These cross disciplinary subjects, and they're fascinating. There's so much high potential, but there's two, there's two vocabularies of discussion to two couple of different, you know, ways of thinking. So be able to commute back and forth between the two. That's, that's a hard that's a hard skill to do. Yeah,
Sebastian Hassinger 42:37
yeah. James, do you think that kind of just begs the question, do you think eventually these all converge -- does quantum information science and up bridging?
James Whitfield 42:46
Yeah
Sebastian Hassinger 42:46
Oh, well, that was easy.
James Whitfield 42:48
I mean, in many ways, even before quantum information science, I mean, Quantum Information Science gives a common play a kind of lingua franca, yeah, it's kind of like Python, or something like or like English, you know, a lot of science, then I think what information science gives that language to bridge the two in ways that are going to be productive and at least well grounded in some concepts. Whereas in condensed matter, you know, you start off thinking about you mentioned Hartree, Fock. Well, hartree fock is also the basis for condensed matter and for DFT, and for quantum chemistry, and all these things end up starting from the same place in terms of what they're doing, and then it diverges very, very differently, depending on which applications are. And so zappy, where's the application is thinking about, you know, the underlying infrastructure mathematically? You know, conceptually, how do these things align is where confirmation science gives us a nice way to kind of talk about these two different areas, in a nice way. If I can plug one thing here, giveaway ideas. And that's what I did.
Sebastian Hassinger 43:46
As many as you want.
James Whitfield 43:48
Great, great, great. So one of the projects that we're working on, and hopefully by the time this podcast comes out, we'll have a few few, a little bit more motion on it. But one of the things I'm really excited, so I'm on sabbatical this year, I've been working on a few sabbatical projects in my spare time. But one of the projects that we're doing is to think about how do we use quantum computers to benchmark what people are doing today. And so we think about the quantum computing quantum chemistry benchmark where we really have the same problems you have for density functional theory. And then the question is, can you reproduce these results? Or if you can't have better results now? How better results next year have better results that you're after? What can you do standard, basic quantum chemistry has been going on for 50 years, 60 years? At all. They get back a number that's meaningful, and how do you actually judge this? How do you set this up? How do you have this the contest is really what we're working on now to invite people to really think about this with us that it's not just saying, here's the right answer. But here's how do we do it together as a community to focus people is
Sebastian Hassinger 44:55
that is the challenge expressing that in encoding You mentioned Python, is that writing the Python to actually run that on a quantum computer or quantum simulator? Or is that trivial? Is it like, is it implementation? That's hard?
James Whitfield 45:11
So I think for me the the motivation for the whole contest idea. I guess, hopefully, I'll do it. Somewhat schools before I do this, you know,
Sebastian Hassinger 45:21
it's fine. Get a website up, James. Just get a website. Yeah, we
James Whitfield 45:25
have. We have one. Yeah. Okay. Well, I'll
Sebastian Hassinger 45:27
give you the link to the show. Yes. It'll be in the show notes. For
James Whitfield 45:29
sure. Exactly. Exactly. Yes, give us motivation. Yes. But yeah, the idea there is that, you know, you want to test out the devices. But the implementation is not necessarily the hard part. The hard part is that there's 150 papers in VQE, sure, or 300 papers of VQE or 50 papers, shoot three papers and VQE. I mean, I don't want to implement this for you. And so if you did an idea, and also I think, even not just implementing it, but you have VQE as an idea, just to think about how much it costs to run rational eigen solver, you know, do How much did it cost? You know, how much how quickly did you converge? How good was your answer? Was your time to convergence? You know, was it a cheap way of getting the right answer? Did you have to use quantum at all just use a quantum qubit to flip a coin and pick out two different DFT functionals? Like? So there's a lot of questions that kind of come up pretty naturally as far as math interesting. But I think just having that question sharply defined. And having some of the best minds come and think about it with us is really what we want to do
Sebastian Hassinger 46:33
is that you sort of think of this in terms of almost like a quantum chemistry version of of CASP of the the the protein folding competition that Davis puts on every couple of years.
James Whitfield 46:45
I think that's pretty similar. I think that that I think that's a similar idea. I would say a lot of the hackathons and Kaggle, things like this are similar. I think a lot of the quantum hackathons happen. I think my goal here is to have it more professional.
Sebastian Hassinger 46:59
Well, and also having I like the idea of having you know, it's a challenge, right? It's like a repeated, you know, having people repeat, you know, attempts at coming up with a better answer for a challenge that can be really, especially in sciences, it can be particularly powerfully motivated. So
Kevin Rowney 47:16
James does this a bit like the there's in the machine learning community, there's this thing called the the, I think it's the glue leadership board or something. It's a bunch of different competitions on natural language processing tasks, and they have been the best known implementation for, you know, cracking a particular NLP task. And then you just really show who's up on the leaderboard or who's leading and how they're, how they're progressing. Is that the kind of concept you had in mind?
James Whitfield 47:42
That's a concept we have in mind. Exactly. Very powerful issue about having. It's usually powerful. I think the hard part is to think there's another really nice one, I think, is good analogies. The Robotics Challenge.
Kevin Rowney 47:56
Oh, yeah. Oh, yeah. No doubt. Right. Right. It's just to show you the current state of the RT, and it keeps raising everybody's game because they can see what happened last year. Right.
James Whitfield 48:05
Exactly. And so that's really the I think that's along the lines, what we're hoping for is that it shows up like that, that you have such a hard technical problem that, you know, you can try it if you're in high school, but like, you know, you're gonna really need to do some quantum chemistry, you're gonna need some quantum computing. Yeah, I mean, I'm not gonna if you know what you're doing. You're not
Kevin Rowney 48:27
Yeah, but for the faint of heart,
Sebastian Hassinger 48:30
do you have? Do you have a name for this yet?
James Whitfield 48:33
James? Yeah, it's the Quantum Computing Quantum Chemistry benchmark. 2023.
Kevin Rowney 48:37
Cool. Love it. It's such fun. Breaking news here. Thank you, James. That's cool. Yeah, that's
James Whitfield 48:45
a lot of people. You haven't invited anybody. By time this comes out at some point going out. At some point, yes. All right. Good
Sebastian Hassinger 48:56
luck on that man. Well, you mentioned, you know, what I know is another one of your favorite topics, which is how to teach quantum information. And your starting point being probabilities. That that makes sense to me. But I know you've thought quite a bit more deeply than that. Are you saying like, at every level, like, Is there is there an optimum sort of starting point? In terms of, you know, age level, or? I don't know, I'm just curious. You You're sort of thinking about education? Because I know you think about it a lot.
James Whitfield 49:29
I think a lot of it there, but also would. That's how I know. So I would say the part that I guess we're on a podcast, so I guess I'll tell you about the stuff I don't normally talk to you about. So I guess, you know, we talk a lot about q 12. And these different K through 12 efforts and K through 14 efforts. I think what I've been doing one of my other sabbatical projects is thinking about how to teach graduate level corn information. So my colleague Lorenza Viola had been teaching this course I think one of the earliest confirmations science graduate courses in the Ivy's. And she gave it to me a couple years ago and I, you know, have notes for decades. And you know, this this last time I taught it last year in the spring, I tried to write notes for every lecture. And so I did my best to write the lecture notes. But you know, I'm okay as a writer, but I'm not that fast. So I wrote my notes, there were decent, you know, proof read them once gave the students and they were like, these are really, really good. You should have given this before class. So we can read these, and then understand and then discuss them. So it's like, Oh, you guys like the notes that much? Fine. I'll write them up. And so been working on this as a project to kind of think about exactly what your question was, how do you teach this the right way, or at least my thoughts about it. And what I find really interesting is that I should finish this also quickly, because I've been paying attention to how a lot of the modern work is coming out. Now. You know, post Nielsen andChuang, if you look at John Watrous, he's giving a really nice set of lectures. And the way he started off his lecture series is also with probability first. He didn't call it that. But like, he starts off with what measurement is of a coin flip or whatever, I don't remember exactly how it started. But when you start with what is measurement in probability theory, what do you feel are your Bayesian or frequentist, whatever you want to feel about it, we have discussion about this. But then we're dealt with philosophy after we finish that, right, this is my way of getting rid of that whole discussion. And not in a dismissive way, either, that saying that, that's good research, fine. But that's not what quantum mechanics is. Quantum mechanics is what you do after you decided those questions,
Sebastian Hassinger 51:27
shut up and measure or calculate the calculus?
James Whitfield 51:32
How we would look at it, but like, I say the same thing with probability. Right? You know, you don't have to think about the philosophy probability, what to calculate what the, what the outcome statistic is, you know, for the T2 test, it's some numbers you put in, you know, ANOVA, that comes back out. Right. And so, you know, it's not really a real question of what does this mean? Just watching? Right,
Sebastian Hassinger 51:50
right? And is the is the goal to put together? Are you to share that online? Are you in write a book? Or both? What's your thinking?
James Whitfield 51:59
Is that the same thing? Yeah. Yeah, I mean, I, what I want to probably do is complete the notes at some point that I'm happy with them, give them people to use in their classes and see if their students are happy with them, take some feedback, round it up. But the the first few chapters are pretty much what I think it should be. And what I started off the book with was kinematics and probability that you do probability theory, we just hold three axioms, the probability the numbers in the vector need to add up to one, they need to be greater than zero. And, and they should all be positive. Yeah.
Sebastian Hassinger 52:34
So but when do you bring in negative probabilities, since that's such a,
James Whitfield 52:39
so my approach to it has no negative problem. Okay, there's Wigner distributions, but I actually I think, would be really interesting project for someone to go back and see what I did in terms of distributions. But I have no idea I did in terms of kinematics of the vector. So this vector has these properties, you have to maintain. And you can go through probability theory, get out market transition matrices, the population, you know, dynamical version of it, we basically have what happens is differential equation, you can get out, you know, normalization, you get out Sharpe distributions, all sorts of things about measurement, all these things happen, that entire kinematic framework you did boost over to quantum and follow the same exact steps. We're gonna start off with the density matrix, and I always use the term probability density matrix. Yes. Because then it makes more sense, because the probability Yes,
Sebastian Hassinger 53:30
yes. As a Yeah, yeah. It's it's
James Whitfield 53:33
probably the density matrix. And then you just look at the kinematics is probably needed to meet the kinematics of the probability density matrix. And that gives you back quantum channels, the Lindblad equation, the Schrodinger equation, unitary versus non unitary evolution, noise, all these things show up immediately it with no context of quantum just kinematically these are the equations that dictate how this has to stay a vector that has Eigen values that are all positive Eigen values that are greater than zero or greater than or equal to zero and eigen values that are the sum of the ones. And that the real, that's the real so that the real is that it has to be Hermitian. Yeah, that is some of the what is the trace is one and then you know, all these things did allow for coherences, all these dynamics, you get back the full structure of quantum mechanics without doing any further physics. Yes, yes.
Kevin Rowney 54:32
Wow. It really interesting. It's so good to hear somebody focusing on the pedagogical concerns. I mean, these are aggressively non intuitive. Concepts. Right? There's so many students that what probability Yeah, exactly. Yeah. Yeah, I get values on that linear algebra and all that complex analysis. There's a lot of stuff under the hood there. So it's, it's good to hear somebody really focusing with with rigor on on decent pedagogy because it's the walk in the park.
James Whitfield 54:59
I also say it's really interesting, like for quantum mechanics, when you go to atomic physics, the first thing you encounter is angular momentum. Angular momentum is so hard to understand without quantum mechanics, and it's like, you really have to go to a merry go round, think about when you throw the ball, which ways you're gonna go. All sorts of like, you know, very basic questions about what angle A victim is, that, you know, are very tricky to do even without quantum mechanics. And so when you go to quantum mechanics doesn't make it easier to understand a complicated subject, the same thing is true of correlation, causation versus correlation, you know, should I use a dis test for that test? Or how to adjust physically significant, you know, all these different questions, statistics show up almost immediately when you go to quantum mechanics, because it's just an extension of probability. So you didn't get past probability by going to quantum mechanics, but rather, it's underlying it. So if you didn't understand it, then you're not going to extend it by popping to quantum medicine interesting.
Sebastian Hassinger 55:54
You know, quantum and AI often get grouped into the same category in terms of technology. And I often sort of wondered about that. But what you're saying kind of suggests, I mean, if you think of AI and ML, current iterations of AI, and ML is being rooted in data science, what you're saying is sort of that, you know, that that's probably statistics that underpins all the machine learning models is is, you know, the same basic tools you're using in quantum information.
James Whitfield 56:24
Yeah, I mean, in many ways, yes. I mean, so they're extended from the tools that you're using machine learning or any other statistical analysis, you can send the quantum. And that's what you know, a lot of the papers are just extending classical concepts to Quantum. And then you get whatever you were doing in probability theory, you can then do it in quantum theory,
Sebastian Hassinger 56:41
you mathematicians too clever. It's I do think, I mean, it's so interesting, James, that you've got this sort of journey from, you know, a deep interest in math, and then almost like, pre med and then turn into chemistry and physics, and then quantum chemistry and then physics. You know, you really do exhibit sort of different modes of thinking, whether you're, you know, whether you're conscious of it or not here, more physics, more chemistry, more math kind of coming out in different different aspects of how you talk about this stuff. It's, it's fascinating to me, because I'm not any of those three things. But I might conceptual understanding often leans on one or the other sort of frame of reference. And it's just really interesting to hear you flipping back and forth between them in a way that's pretty unique, I think,
James Whitfield 57:35
yeah, I can, I can, I can point to my research group. I think one of the things that I really had a lot of fun, said at Dartmouth, a lot of people come in, they come for whatever reason, they didn't end up at Stanford, or, you know, Caltech or whatever, they're at Dartmouth. So it's like, right, come on, come on down, guys, we're gonna make you the best scientist you can be. And I've had some really good scientists who are about to leave my group. So really good scientists in my group. But because they're all in my group, they all getting indoctrinated in this, like, mix of chemistry and mathematics and confirmation science and, you know, this kind of explore this area that no one's in, just have fun. Just kind of go look around and see, what do you find in restaurants today? And like, it's, you know, we're in Hanover, New Hampshire, it's like, relax, we're not gonna compete with Boston, or, you know, you know, can't go go against the, you know, the entire algorithms team at Amazon, right, we're gonna kick back and have some fun, and you know, talk about it.
Kevin Rowney 58:30
So yeah, nice gig. It's really cool.
James Whitfield 58:33
Yeah, and so we have a real good mix of talents and semi groups. So it's a lot of people who some of them focus on math, some of them are trained to be quantum chemists, some of them are one of my graduate students is really, really good at quantum chemistry. You know, he's, he's, you know, ready to go become a coder or a quantum chemist. And so we got, yeah, we got alumni headed off there, one of them will join us at AWS, then nonetheless,
Sebastian Hassinger 58:57
very cool. But do you think and I'm mindful over time. So here's your wrap up question. Do you think I mean, you mentioned, you know, quantum information theory, or sciences is sort of lending a lingua franca was the term you use? Do you think that eventually this does, you know, software eats all of these disciplines of science as well, and we end up being, you know, we end up with scientists being coded or writing code. And that code happens to be solving problems in chemistry or solving physics problems or solving, you know, other other scientific problems through writing software?
James Whitfield 59:31
That's a nice question. I like that question. I think not. A good my argument for I think this question touches really nicely on this whole zeitgeist of AI and people worried about how they're going to take our jobs and go do this, that a third. My thing is, there will always be smart humans, and I don't mean that as you know, up or down, you know, whatever it is this to be a bell curve. There's gonna be somebody at the bar and somebody have it right. There's kids that are at the far end of that will always figure out how to use it tools, and how to make better versions of the tools figure out how to play with these things in ways that we haven't thought of. And I think that's really where you get creativity, you get whether or not you want to use computer, a lot of scientists don't use computers. So they still fantastic scientists take entire journeys inside their head, building mathematical structures, they don't, you know, don't bother to code it up, and then they give it to someone else who codes it up. And I think there's a lot of space for people who are intelligent to just get together and think of more intelligent things, the tools that are coming out now will only help them do more, they'll be able to get back to the tool and whether they don't write any code at all, they told you at GPT to write it up. Here's my idea to code this thing up. And then they could do 10 ideas in a day rather than having to spend all day to learn C Plus Plus and you know, learn Python, they can just say hey, here's my code, man, I want you to cut up this differential equation for me. And then that's it can learn something else and they can just write
Kevin Rowney 1:00:53
great perspective it's an amazing time to be alive in many ways.
James Whitfield 1:00:59
I like spellcheck
Sebastian Hassinger 1:01:06
super useful and glasses.
James Whitfield 1:01:10
That was also fantastic.
Sebastian Hassinger 1:01:17
That's great. James. Any anything any last thing you want to plug or any last thing you want to words want to leave us with?
James Whitfield 1:01:26
Think about this. No pressure, not pressure. It's all here. It's all here already. Yeah, I don't know. I I wish I had better than I don't know.
Sebastian Hassinger 1:01:36
No, we had we had one right ending, I just wanted to give you one last chance because I kind of asked the last question knocked out of the park
James Whitfield 1:01:44
was at the interview and they're like should have a question.
Sebastian Hassinger 1:02:34
Okay, another fantastic conversation we had there, Kevin, I really, really liked James's view on views on where he thinks quantum computing will will first sort of provide practical advantage in quantum chemistry, it's not sort of the standard view that he adheres to. Right. Quite quite
Kevin Rowney 1:02:53
so I mean, look at this whole distinction, right, between, you know, finding a ground state of the systems versus looking at the time evolution of them is a distinction that is not I think commonly talked about and, you know, it really I think elucidates whole new domains where perhaps quantum computation could make gigantic inroads and perhaps even pioneer very novel new forms of science and, and, and applications and in the real world. So powerful insights, I
Sebastian Hassinger 1:03:20
thought, yeah, and his reasoning really resonated with me, because, you know, as he pointed out, classical Computational Chemistry has really been focused on grounded state estimation, grand state optimization for quite some time, and just really, right. It's a fairly long time. And so there's really powerful tools to to get good results in that in that way. And so it's unlikely that these super early stage quantum computers are going to outperform the best sort of classical approaches that that we know today. Right?
Kevin Rowney 1:03:52
Yeah. But there could be all sorts of novel problems out there that have just never been investigated before. Because they're so challenging. They could be so so it's resolving, I think, with better clarity, optimism in a particular area, grounded optimism, and then skepticism of other areas where, you know, not, not really I think there are a lot of room for progress. So yeah, you're right. I
Sebastian Hassinger 1:04:14
mean, it's the it's difficult as, as James pointed out, it's difficult for people to bring to bear sort of their scientific inquiry in that way with quantum computers, because that's not what that's not their sort of starting point. I think. It speaks to James as sort of novel collection of different disciplines and different sort of frameworks for understanding that he's taking this sort of, you know, sort of blank sheet of paper kind of approach to what what are these machines potentially good for? And I'm hoping I mean, it was great to hear about is quantum benchmarking event that he's planning this summer. Hopefully that's cool. Actually. It really does. Yeah, it really does. So we'll definitely have a link to his site in the show notes. When this gets pulled This did and we'll keep you up to date. Maybe we can do some kind of special episode on the outcome of the first. Yeah, that'd be great.
Kevin Rowney 1:05:11
All right. Awesome.
Sebastian Hassinger 1:05:12
Well, thanks, Kevin. See you next time.
Kevin Rowney 1:05:15
I met see them. Okay, that's it for this episode of The New quantum era, a podcast by Sebastian Hassinger. And Kevin Roni, our cool theme music was composed and played by Omar Costa Homido. production work is done by our wonderful team over at pod phi. If you're at all like us and enjoy this rich, deep and interesting topic, please subscribe to our podcast on whichever platform you may stream from. And even consider if you'd like what you've heard today, reviewing us on iTunes and or mentioning us on your preferred social media platforms. Were just trying to get the word out on this fascinating topic and would really appreciate your help spreading the word and building community. Thank you so much for your time.
Creators and Guests
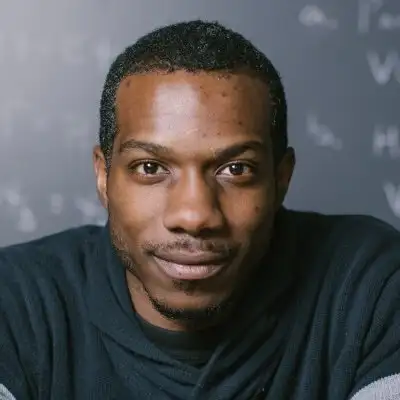
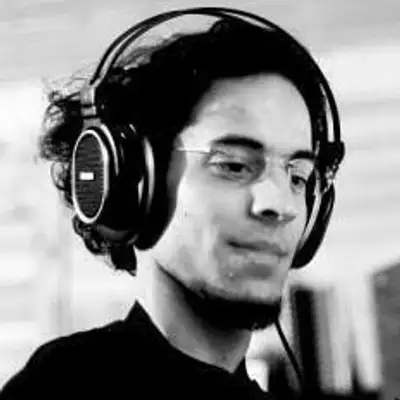
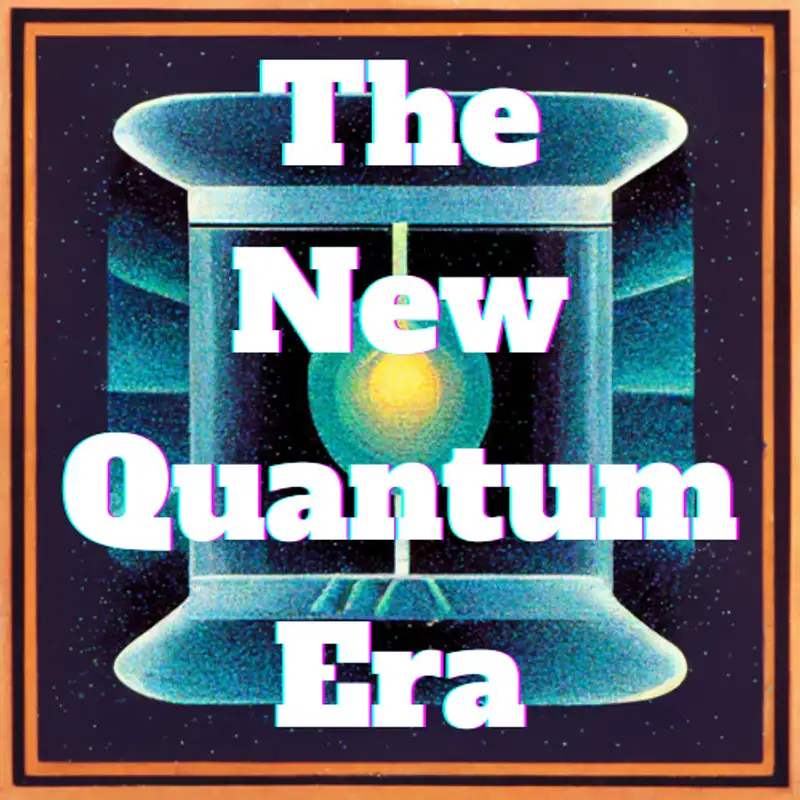