Modular Quantum System Architectures with Yufei Ding
Download MP3The New Quantum Era, a podcast by Sebastian Hassinger.
Kevin Rowney:And Kevin Rowney.
Kevin Rowney:Welcome back. Hey. After a brief hiatus, I returned to the podcast. Hey, Sebastian. Hey, today today, we've got a really interesting interview with professor Yufei Ding, who's a associate professor at UC San Diego.
Kevin Rowney:And she's got a kind of a cool background. It's sort of at the intersection between theoretical physics and and computer science. So, I I think this is gonna be a a good one, Sebastian.
Sebastian Hassinger:I agree, Kevin, and welcome back. Good to have you back. Thanks, man. I met Yufei, at a panel at IEEE Quantum Week in, Bellevue, last September, which is a terrific event overall. The whole week was great.
Sebastian Hassinger:The panel was academic quantum devices and their vertical stacks, status quo and future roadmap. A little verbose, but it was super fascinating. It was a combination of people working on hardware control stacks that are open source and the software control stack sort of above that, the controls as well as compilation optimization and then these sort of system architectures. It was a really, really fascinating conversation, and I was really struck exactly by what you said, where UFAY is sort of coming at this, through an intersection of sort of computer science, computer architecture, and the the quantum computing side of things. So I thought that was really fascinating, and I'm really looking forward to the conversation.
Kevin Rowney:So awesome. Let's dive in.
Sebastian Hassinger:Welcome back. So, we're joined today by Professor Yufei Ding, from University of California, San Diego. And Yufei, thank you very much for joining us. If you could briefly introduce yourself, that would be great. Welcome Yufei.
Yufei Ding:Thank you. Thank you. Like, I really like, thanks for the great opportunity. Hello, everyone. I'm Yufei Ding.
Yufei Ding:I'm associate professor at the computer science and engineering department of UCSD and my research field is mainly like 2, two reactions and one is the machine learning system. The other one is quantum computing systems. And, you may wonder why I study these two research areas and I want to give you some like some kind of introduction to my research background. So I get my bachelor and master degree in physics and then like I asked page 2 computer science and doing my PhD there. And after graduation I get my faculty job.
Yufei Ding:And at that time I was thinking, okay, how, what kind of research direction I should, pursue? And one of the direction is like continue or continue of my PhD study, which is a machine learning system. And it's a hot topic. I think it's giving a lot of impact. And another direction I'm thinking of is you really because faculty, I think 1 or 2, like maybe 2 research is is try to keep your abreast and also I still keep your, keep your doubts.
Yufei Ding:So the other research direction I was thinking about that time is about quantum computing. And I think quantum computing is rising at that time. And I also think like my grand, my background is, is pretty unique to like for, to explore that direction. So that's why I studied that direction. I think, I joined as a at a very good time.
Sebastian Hassinger:Did did you have a physics background in addition to the computer science? Yes. Studies? Yes.
Yufei Ding:Yes, yes, bachelor and master degree in physics.
Sebastian Hassinger:Got it, okay.
Kevin Rowney:So interesting. And often, Yufei, what we ask our guests on the podcast is, was there any particular moment or influence that powerfully influenced you in the past to take the choice to embrace quantum computing as a focused area of study.
Yufei Ding:Uh-huh. Uh-huh. So I still remember, like, why I first, like, study, like, physics in my college. One of the most interesting and really inspiring course is a quantum mechanics course. Mhmm.
Yufei Ding:And I still remember at that time like the the teachers tell all different kind of phenomenons because like how Einstein and a lot of like physics experts, like at that time I were arguing about like why this could happen and what are the fundamental challenges, what are the fundamental series. You can feel like sometimes, like, we always think, like, if that happens to me, if I was born at that time, can I, like, really No? No. No. A crazy kind of sense.
Yufei Ding:So sometimes you feel like, okay. Because people sometimes feel some kind of research. They feel like just because, like, you are not born at that time. You are born that time, maybe you can also create it. But some kind of serious.
Yufei Ding:You wonder whether you even like you are are like born at that period of time, and you have that opportunity while you're really like drawing this kind of tools. Because it's so challenging, it's so transport transformative. And you always wonder like because like how can we make this kind of trans transformative change to being capable? What kind of research background you need to have to see this well, this kind of new research as an incremental research. Because in my field, my feeling is everything after you have good enough or like good enough background or like understanding of the field, everything can be incremental from some perspective.
Yufei Ding:But if you are missing some technologies or missing some of the knowledge, it won't make you like to feel like it's so so all of a sudden is too like, the gap is too huge for Right. Going. But yeah.
Sebastian Hassinger:So you're you're drawn to the really deep unknown questions then is what you're saying. Yeah. That's great.
Kevin Rowney:Really interesting. And so be more specific, please. I mean, which which big frontiers, have drawn you in? You you that you think are the most in need of calling out for researchers like you to make a contribution towards?
Yufei Ding:Okay. So so like for my current research is about quantum computing system. I think system is super important. And the reason is if you look at quantum hardware, you will only have like a few cubits. It's hard to say like what kind of system you want to build.
Yufei Ding:And computer science if you look at it or especially system research compared to physics. Physics is try to really understand how their the nature is working. But computer science is to harness this kind of nature to make it useful, okay? To make it practical. And we need to build really complex system to leverage everything, to make them work uniquely and also smoothly.
Yufei Ding:So so there are a lot of design points here especially once the scale getting getting larger. So I think that's a beauty of the system research. And I feel like my research is very important is because like the is also like depends on the involvement or the advancement of the hardware development.
Kevin Rowney:Yeah. Really interesting. I mean, these pragmatic concerns about system architecture, I mean, we really are at the frontier. We've only seen a couple of, of podcast interviews here that really touched on this with with depth, but it feels to us like the pace of that research is increasing. Right?
Kevin Rowney:It's a very creative time, in in that in that domain of research. Very interesting.
Yufei Ding:Mhmm. Thank you.
Sebastian Hassinger:Yeah. I mean, that was, if it when I, participated in that workshop at IEEE IEEE Quantum Week where you presented your work on on system architect quantum system architecture, that's really when I started thinking, oh, we're gonna have to have her on the podcast for sure. Because I thought it was so fascinating. If you look at at any particular effort to build cubits, they may be thinking about scaling up a single node. They they may be thinking about, scaling it to multi node, but they're only thinking within their own modalities, super conducting cubits or trapped on cubits or whatever.
Sebastian Hassinger:You're really thinking across modalities, and you're thinking about what role in a system architecture a particular type of qubit may be able to play. Is that is that right?
Yufei Ding:Yes. Exactly. So I want to, highlight a little bit of this kind of of function, like decoupling in classical computing. So if we demo, like we you already have memory and processing like the CPU and GPU, and we have the memory system. And the reason we want to decouple them is we only want to focus on some of the functionality at each component.
Yufei Ding:For example, while you have memory, like you can focus on the storage, like, the memory size. Yeah. Usually we do not do not directly do computation on the memory.
Sebastian Hassinger:Right.
Yufei Ding:Because, yes, you can do that, but you already do some simple computations. But that major functionality for memory is loading, storing. And that like because of, and then the processing unit is forcing on different kind of arithmetic Right. Control processing. And this kind of function decoupling really create a lot of research opportunities and try to make the system better.
Yufei Ding:Okay? And it's similar to like how we work as a human being. So if you think about one person, you need to learn all different kind of stuff. It's super hard. So that's how we have different roles.
Yufei Ding:I think similar things can happen in quantum computing and make our system scaling better.
Sebastian Hassinger:Interesting. And that's interesting too because in that way you're you're taking what may be, a weakness in a particular modality of Qubit in one dimension and and focusing instead on the strengths in another dimension? As you said, it may be ideal for storage and not so great for computation because it's not as fast or whatever. Is is that kind of the sort of the driver behind the thinking? Yeah.
Yufei Ding:Yes. Yes. Exactly. And sometimes it's also like depends on what kind of applications we want to do. Right.
Yufei Ding:And and that some kind of applications may for example, like we we can have different kind of data encodings. We can use like cubits, cuDits, and continuous variables of different ways of encoding. And for different problems, we may or like even for one problem, different parts of it may require different kind of encoding. And that also requires, like, different kind of functionalities in your hard work, on your hard
Kevin Rowney:work. Interesting. Interesting. And I think another big dimension of your research is around, yeah, algorithms and systems for the interconnection of the 2 remotely separated quantum computing systems and how they could perhaps interact with speed, efficiency, and low error rate. Could you fill in our audience a little bit more in detail how how those systems that you proposed to work?
Yufei Ding:Okay. Yes. Yes, definitely. So if you think about it, current the quantum hardware is trying to scale it up. But while scaling up, there's a lot of like fabrication challenges.
Yufei Ding:So if you put too many qubits on one chip, they're like 0 could some like, the fidelity of each qubits, like the gate errors could increase. So like one way they are thinking of is using, for example, chiplet to interconnect small chips and then using distributed quantum computing to connect like a lot a larger like chiplets. So this can kind of like create this kind of hierarchy hierarchical like structure of quantum hardware. Okay? And in that case, you may wonder like it's very similar to like what we have in in classical computing from single node, our single call to multi call to distributed classical computing.
Yufei Ding:So very similar to that from that perspective. But then the problem is if you have application Okay. Yet like you have to distribute your application like an executed locally on each of them. Yeah. Sometimes you need to communicate our communication among different kind of chips, and the communication can become the performance bottleneck.
Yufei Ding:Okay? And then like the system is try to minimize this kind of communication bottleneck. Okay? And our like, oh, so usually one of their strategy is very similar to the classical computing is try to minimize the number of remote communications you need to do or remote gates you need to do in quantum computing. And this is done by like some graph cutting like graph cutting like, methodologies or techniques that we are looking a little bit ahead.
Yufei Ding:Because for quantum computing, sometimes like sue one of the communications, you may do multiple gates and that is based on the stress of quantum computing.
Sebastian Hassinger:Right. Okay.
Yufei Ding:Yeah. And that is kind
Sebastian Hassinger:of I was about to ask. Yeah. How does entanglement and superposition actually affect the standard sort of classical perspective on that, on that distributed challenge. Yeah.
Yufei Ding:Exactly. So it's kind of like a quantum fan out. Okay. Try to do that effectively. Okay.
Sebastian Hassinger:Interesting.
Yufei Ding:Mhmm. Mhmm. So your requirement computing, we know that we cannot clone, and so we cannot easily do the fan out. But they have their own way of doing this kind of cat, like cat mode, like cat cubits. Yeah.
Yufei Ding:In this kind of cat entanglement states, try to do this kind of quantum found out and do the optimizations. Okay.
Kevin Rowney:Really interesting. Yeah.
Sebastian Hassinger:That's great. And, when you when you work on these these sort of concepts of system architecture, are you combining, you know, obviously, you're using simulators for for quantum circuits at this point because you need sort of more perfect cubits which don't exist yet. But are are you also combining, like, simulators of quantum communication as well? So that because I mean, obviously, part of the the the strategy of distribution requires flying cubits in addition to computational and storage cubits. Right?
Sebastian Hassinger:Is that is that how you sort of try to work on these things?
Yufei Ding:That's a very, very good question. So currently, like our simulators feel like simple, but we are trying to push in, push it forward using different kind of ways. So what I can tell you is usually, like, we look through the physics papers, try to understand, okay, what is a remote, like, fit all the fidelity of the remote gate. And try to get information. And then, like, we want to, like, really simulate how that how that remote gate is different from the local gate and use that information to do our optimizations.
Sebastian Hassinger:Interesting.
Yufei Ding:Yeah. But our simulator is general or like is extensible. So you will we can also study like if you can improve your fidelity like of the remote gates. And after you like physically improve that that link, what is kind of the performance improvement on the system level? So that's why I'm also thinking like the system research is kind of guiding, could could Right.
Yufei Ding:Properly guiding the, guiding this kind of hardware research before we even like push it forward, try to spend a lot of money to push some of the component into into a better shape because usually we can have different kind of choices. You can improve the links. You can improve the, the probability of the success. Yeah. You can improve of different kind of factors there.
Yufei Ding:But which factor is the most important?
Kevin Rowney:Oh, I see. So the the the simulation agenda that gives you perhaps, you know, numerous angles of research to allow you to figure out which of the various physical manifestations have to be perhaps pushed the hardest to get to a real a real life system. Yeah.
Yufei Ding:Yes. Yes.
Sebastian Hassinger:I see. That's cool. So does that in your mind, do you think that there is before we get to those perfect Qubits, the the fully, you know, fault tolerant quantum computer, Do you think there may be interim steps or error mitigated or or otherwise fault resistant, but not fully fault tolerant that might actually yield a a useful result in this kind of distributed architecture?
Yufei Ding:Yes, definitely. I think that is a way around. So like, so, so I think there are 2, like, research directions we can think of. And why is practically, like, before we can have the quantum arrow of of a tolerant quantum computing with really large scale quantum error correction code being applied. We can think about quantum like, this kind of error mitigation and maybe partial error correction code and how to apply that.
Yufei Ding:For example, we can only, like protect some of the logic qubits, which is frequently used and less production on those qubits is less used. But we also need to ensure that error information from the bad qubits do not propagate to the good qubits when they communicate with the children. So what kind of strategy we can use? Yeah. We need to have a lot of system, simulations.
Yufei Ding:Try to give a and try to give a lot of like good ideas, try to explore that direction. And others on the other side, I see it also be very interesting to work with physics to understand, okay, what is extreme between software like logic qubits and hardware logic qubits. So for example, the topological like quantum qubits, I can be as ideal like hard wall, like protective.
Kevin Rowney:Right.
Yufei Ding:Right. Okay. And like for example, like freezing our superconducting, superconducting like cubits today and apply the error correction code on that or to a larger scale, this could be extreme of the software protected cubits.
Sebastian Hassinger:Right.
Yufei Ding:While in the middle is the best one to design. I think, yeah, that could leverage our hardware like properties or innovations as fuel like effective. So so I think it it must be something in the middle. Yeah. Try to go through, like, go deeper in that direction being like, try to explore different kind of system, strategies.
Yufei Ding:Try to understand, working with the hardware people, try to understand which part of the cubits can be tuned and which perspective can be tuned. And giving a full knowledge of that part can help us to meet in the middle. Okay. So that is I think another very important
Kevin Rowney:perspective. Ambitious ambitious agenda. Very interesting. I I wonder I mean, I I I'm tempted to now pivot to a separate and very interesting domain of of quantum computing research. And you seem like an ideal party to talk to this one about because, yeah, there's, of course, a broad range of of both optimism and pessimism about quantum computing.
Kevin Rowney:Yeah, we we are we're believers. It's so interesting and so powerful. But, you know, the specific domain, right, of the intersection between machine learning and quantum computing, you know, many people, of course, just dismiss it as as hype, but there's also so much room for optimism. I mean, numerous, commentators, you know, Scott Aronson and others have, underlined the need for rigorous skepticism about these algorithms. What is your level of faith and optimism, right, about the future of how quantum computing could perhaps, transform future machine learning research?
Yufei Ding:Mhmm. Mhmm. That's a great question. So first, like, let's think about quantum computing for machine learning, and then we can talk about machine learning for quantum computing.
Kevin Rowney:It's very, very well.
Yufei Ding:Yeah. So quantum computing for machine learning. So why people are super interesting about it? Because, like, if you think about it, why NVIDIA? Like NVIDIA, like today is super like a big company, and it's a big drive for for for for machine learning because machine learning is a killer application.
Yufei Ding:Yeah. Once you have this killer application, it has a lot of usage in different kind of domains. People like even like you you claim is a hard work accelerator is only useful for some of the applications. As long as that application is very useful, then like it become super, super important. Okay.
Sebastian Hassinger:That's right.
Yufei Ding:Yes. So that's why like people have the dream, have the passion to push quantum computing for machine learning. And there are something like that drives people to think in that way. One of the reason is nowadays the quantum computing is still noisy, but machine learning has a lot of tolerance for noise. So that's why people feel maybe we can do that because, like, you're already wasting everything is a black box.
Yufei Ding:Yeah. Suddenly, it just works. So potentially maybe it could work in some way as long as we can find the right way to encoding it. So that's why like, I feel like Cirritus is more perspective in that direction, but they are pushing in the right way. Because sometimes we need to understand what kind of model we need to use and what kind of data encoding we can have here, what potentially of the speed that we can have there.
Yufei Ding:But sometimes even like the serial theory cannot prove it could work, and suddenly it could work. We can still have the hope there. I feel like we are pushing it in both direction, empirically and theoretically. Okay? Because it's super great performance for machine learning, they're like quantum computing will have a huge market.
Kevin Rowney:Right.
Yufei Ding:We'll have huge potential. Yeah. So that's why like people are really push it forward. Okay. I'm also a little bit conservative, but if I have time, if I have a student or like after like I I study like the 2EC, I would definitely put him, if like him or her on that direction.
Kevin Rowney:Oh, yeah. Yeah.
Yufei Ding:If they are like also inspired to to explore that direction. Because whilst that can be con confirmed, I think that will be huge contribution.
Sebastian Hassinger:Huge. Yeah.
Yufei Ding:It's super impact like, huge impact.
Sebastian Hassinger:Okay. Interesting.
Yufei Ding:And that is one direction. And the other direction is machine learning for quantum computing. I think that one is very natural. And, because a lot of this kind of system optimization, error correction, error mitigation, the system designs, compilers, architecture designs, everything can be regarded as optimization problem and machine can definitely be helped there. Okay.
Kevin Rowney:Doubtless. I mean, there's so many powerful research results already obtained on this path. There should be no question. Skeptics there perhaps should quiet.
Sebastian Hassinger:Yeah. That's a really good point. So I mean, that's that's a great, sort of illustration of why, these this kind of system approach is important because you're describing 2 different technologies really and how they can interact in a systematic way. You know, throughout this conversation, I just keep on thinking about, the way that classical systems have been architected, really starting from, you know, the the man who who gives his name to the architecture, the Von Neumann's, efforts at the Institute For Advanced Studies. And there, I think, you know, a lot of what the work that came out of there was in response to the fact that they were using, war surplus components that were, very low quality.
Sebastian Hassinger:They were having to deal with noisy, circuitry, circuits that were prone to failure, and and also, limited sort of understanding of of even the problem space they were going after, right, beyond just the sort of immediate computations for physics experiments. So do you sort of see a parallel there between I mean, are you trying to create the Yufei Ding architecture for for quantum systems?
Yufei Ding:That is very ambitious. So but So Yeah. Definitely, like, I'm trying to follow a lot of likes of so so one of the thing, like, I have in mind is usually, like, the philosophy, like, we have in classic computing should still apply here. Because that is really we have thinking about for many, many years. Yeah, but the detailed methodology and approaches could differ.
Yufei Ding:So for example, like in classical computing, when we think about architecture, you only have 2 parts. And one is the ASAT, the in of the, the instruction architecture stat, which is the interface between the software and the hardware. And then we have the microarchitecture, which is kind of as implementation of this kind of SI. Okay. We have different ways.
Yufei Ding:And we'll look at the SI. Usually we have like the way we do data encoding. And currently, like we are mostly think about qubits. But in classical computing is most of bits, but we also have different kind of precisions. So for example, you have you have float, you have double, you have like integers, you have different ways.
Yufei Ding:In quantum computing, we have similar like con continuous variable. We have cubits. We have cubits. And then we have a set of instructions. Like in in in in in classical computing, we can have the arithmetic operations.
Yufei Ding:We have the control flow operations and we can have the like memory operations. Yeah. If you look at quantum computing today, we are not really having a lot of memory operations because we only have 1 layers of computation. So memory is also the compute unit, but later on you may have more like modularity like, like computers. Now we may have that, that components.
Yufei Ding:And mostly like at the beginning of the quantum computing is is the design of this kind of arithmetic operations. Like the 1 qubit and 2 qubit gates. Okay? And that is from the universal, like what kind of gates are good enough for the universal computation? But nowadays, that is extended.
Yufei Ding:So like not only is it universal, but in terms of the performance, what is a good like instructions that we want to have there? And then the other one is a control, like, control flow operations. Like, in classical computing, you have if else, you can have while. Yet in quantum computing previously, like, when we first have, like, is the earlier version of the IBM and Google machines, they do not have their, main circuit measurement. So they do not really having this kind of control component.
Yufei Ding:But if you think about that, it's kind of, you look at the instruction at the beginning, you'll walk us, you may want to have something like that. And that also gives you the capability of doing error correction code error making.
Sebastian Hassinger:Right. Feed forward and yeah. All of the That's all the associate.
Yufei Ding:Yes. Yeah. And and and
Kevin Rowney:and Ansel, like, Qubit's, member store as well. Yes. So we've made
Yufei Ding:That huge.
Kevin Rowney:Important differences. Yes.
Yufei Ding:Yes. Yes. Exactly. So so if we like, my key point is if you look at the classical computing, examine each of these components, and try to think why they are created and how they are created. What are the research problem?
Yufei Ding:You usually have the same strategy, same problems in quantum computing. And sometimes the problem is more complicated. For example, I I want to go back to the memory operations. In in in classical computing, we have the memory. We have to load from the memory to the processors to do the computation.
Yufei Ding:We do not directly have that in quantum computing, but we have something similar. That is because usually the instruction you can get here can only, for example, 2 qubit gates can only be applied to to neighboring data or like data, qubits. So then like if you are applying a a gates on 2 file way data Right. Qubits, then you have to do the routing. So this kind of routing can be regarded as one of this kind of memory loading operations.
Yufei Ding:Okay? And so similarly, even seeing bugs are like in classical computing, we have a big jump to like to from creating this kind of scalar computing to vector machines. Okay? And the vector machine, one of the key idea is a lot of cubits, a lot of bits, they may want to do the same computation instead of like directly fetching a a one computation, we can fetch 1 instruction and do a lot of computation.
Sebastian Hassinger:Same thing. Right. Pipelining.
Yufei Ding:Yeah. Yeah. Similar. Yeah. A lot of ideas.
Yufei Ding:Can we have that in in quantitative? Because the control is not overhead. Okay. So again, like my key point is if you examine the whole strategies, if you have a good understanding of the architectures, you'll find that a lot of philosophy will drive you further. Okay?
Kevin Rowney:Yeah. No. It's so powerful. It it very much builds on recent results out of at MIT and Harvard. They're sort of like the vector parallelization of multiple qubit operations, and and those same memory store issues.
Kevin Rowney:But they haven't they haven't explored the connection of, you know, multiple remote systems. So, yeah, this is rich, deep material. Really, really interesting.
Yufei Ding:Yes.
Sebastian Hassinger:So I guess the the last question I have for you, Yifed, this is terrific. Your work is really grounded in what's actually being built in the labs, in the experimental labs. Right? It's you're taking a theoretical approach, but you're grounding it in what can be built and what what potentially can be realized. Do you see do you get, I guess, a good collaboration with the experimentalists, or do you think there needs to be a better mechanism for for the co design between the theory and the experiment, you know, in in the industry as a whole?
Yufei Ding:Yes. Definitely. I think, like, co design try to, like, let computer scientists and physics working together will be, like, a big plus. Yet, it's definitely something we need to do. And one of the reason is because we are the beginning, like the interface is not clear.
Yufei Ding:Actually, the interface should be created together with the physicists and the computer scientists. And, so so that's why I'm always exploring like this kind of collaboration opportunity with physics, try to understand their demands and try to understand what kind of hardware they can build, what kind of components or parameters they can do, what kind of thing they can do and then like you try to build our system upon it. Yeah. And try to understand. So so I think that is also called a a a digital twin of the hardware.
Yufei Ding:Mhmm. And which part can be tuned. And then, like, build a system to really evaluate this kind of digital twin before, like, we spend a lot of money to, to to tune each of the component. I still hope to really shortening, like, how we really get out get to our final stage.
Kevin Rowney:I I wonder if you say I mean, maybe it's just a wrap up question here, and I, please forgive me if I'm being too direct, but I'm I'd like to if you could try to speculate in a concrete way. I mean, given all this this really interesting and vibrant research going on, I mean, at at what point within our our near future do you think a a real, like, you know, multi tier quantum computing system could we see, bring bring actually to market? I mean, is this a is this a 5 year, a 10 year, a 50 year agenda before we see a a real practical system?
Yufei Ding:I think maybe 10 years is a good, good timeline to see some practical things. But I think in 5 years, we can really see a lot of near term milestones. So for example, we're already seeing like how QEC can scale up at at from from the Google experiments and from the Yield's experiments, we first time have can see a lot of Kubernetes to break even. And I can tell you like a lot of business, they feel like the the the advancement has been much faster than what they expected.
Kevin Rowney:Right.
Yufei Ding:Actually.
Sebastian Hassinger:Very true. That's fantastic. This has really been very, very Well, that was a really fascinating conversation. I really enjoyed it. I hope you did too, Kevin.
Kevin Rowney:Super cool.
Sebastian Hassinger:Yeah. I mean, I think what Yifei is doing is really in the context of sort of the self consciousness of trying to reconstruct some critical stages of the development of classical computing. We mentioned Institute For Advanced Studies at Princeton and Von Neumann where you sort of did the groundwork for what now is considered Von Neumann architecture, which is, you know, absolutely ubiquitous in class Foundationally.
Kevin Rowney:Yeah.
Sebastian Hassinger:Right. And she's sort of taking the learnings from that, and trying to find how it applies to to the current context of of quantum computing and the sort of, you know, the near future of quantum computing, which I thought was really fascinating. I mean,
Kevin Rowney:so cool. I mean, you get the sense that really these days right now, we're living through an era where, like, just fundamental principles of the future, right, for quantum computing are getting explored and defined, you know, week by week. Really, really fascinating view on the the frontier. I thought I thought it was really, compelling also to hear, you know, her overall research agenda and entire, you know, simulation framework for these more in-depth and more well defined system architectures to allow practitioners and experimenters to explore what are viable outcomes. I mean, you know, the rise of, you know, classical computing and parallel architectures, there was long before the advent of real physical systems that the simulation agenda was Right.
Kevin Rowney:Profound, right? And how to map out the best ideas. Right. Dispose of the ones that were just fantasy. Right.
Sebastian Hassinger:Right. And we should bring that to bear to the current landscape of of differing modalities of cubits, super early stage quantum communications technologies, and and as you say, creating a framework, an extensible framework where you can sort of model out, like, if we use trapped ion in this way and we use superconducting in this way and we use, neutralabs in this other way, can we combine the strengths of those different modalities into something that is a quantum system architecture, which I think is super fascinating.
Kevin Rowney:So fun. It was also, I think, fascinating to, you know, ask the difficult questions about, you know, the the skeptics point of view, around the possible hype that people feel exists in the, you know, the intersection of quantum computing and and machine learning algorithms. I mean, obviously, there's no question ML has a immense and, long powerful influence already, right, on quantum computing.
Sebastian Hassinger:But Right.
Kevin Rowney:The other direction is is there, some sort of possibility that, quantum computing algorithms could be pertinent to future machine learning advances. I like your point of view is that, yes, semi skeptics do have a reason to be, you know, doubters. However, should there be a finding in that domain, it could be totally profound, totally transformative.
Sebastian Hassinger:Right. Right. Because of the breadth of the impact of ML across various verticals, various use cases, if there was some kind of generically applicable quantum advantage to some portion of of machine learning that could potentially have impact across some giant swath of that that sort of, solution space. Yeah.
Kevin Rowney:Yeah. Yeah. Very interesting. Really good time. Yeah.
Kevin Rowney:Okay. That's it for this episode of The New Quantum Era, a podcast by Sebastian Hassinger and Kevin Roney. Our cool theme music was composed and played by Omar Costa Hamido. Production work is done by our wonderful team over at Podfi. If you are at all like us and enjoy this rich, deep, and interesting topic, please subscribe to our podcast on whichever platform you may stream from.
Kevin Rowney:And even consider, if you like what you've heard today, reviewing us on iTunes and or mentioning us on your preferred social media platforms. We're just trying to get the word out on this fascinating topic and would really appreciate your help spreading the word and building community. Thank you so much for your time.
Creators and Guests
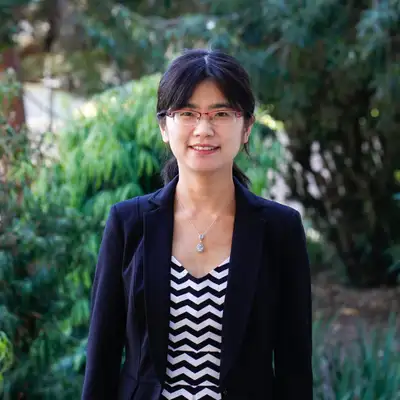
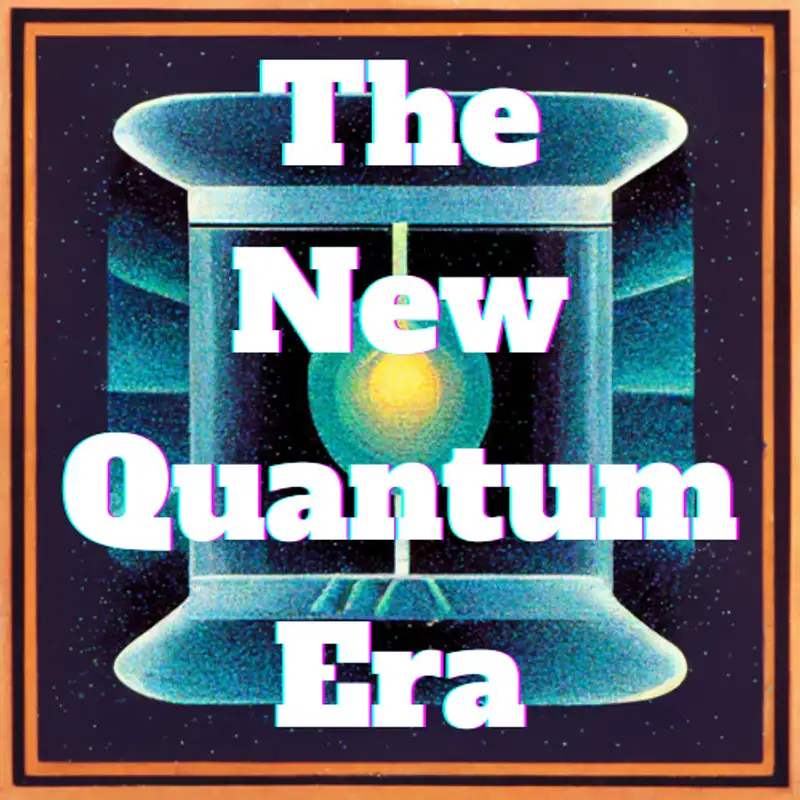