Aspiring Quantum Chemist with Professor Lin Lin
Download MP3The New Quantum Era, a podcast by Sebastian Hassinger.
Kevin Rowney:And Kevin Rowney
Sebastian Hassinger:Welcome back to the podcast. It's Sebastian flying solo again, and I'm back with another episode from my trip to Rensselaer Polytechnic Institute in Troy, New York. This week, we'll be talking with professor Lin from UC Berkeley, who's a professor of mathematics, but the conversation and his career focus, his research focus is really on computational chemistry. Professor Lin takes us through some of the foundations of computational chemistry through the his transition from sort of mathematics to, to exploring the possibility for, extending computational chemistry and then applying quantum computing to that field. So it's a really interesting sort of overview of his work and of the the recent advances in the field and the potential for combining classical machine learning methods for computational chemistry with new and emerging methods using quantum computing.
Sebastian Hassinger:So I hope you enjoy, and let's take a listen. I am joined today by a very special guest, professor Lin Lin from University of California, Berkeley, where he is a professor of mathematics. And, thank you for joining me today.
Lin Lin:Thank you, Sebastian. It's a great pleasure to be here.
Sebastian Hassinger:We're here at RPI, at the ribbon cutting of the quantum computer from IBM that they've installed on-site. It's been really great. All the things that have been going, all the events and everything and talks, But you and I have had a bunch of really great conversations.
Lin Lin:That is wonderful.
Sebastian Hassinger:Yeah. And I'm looking forward to sort of sharing, like, recapping and sharing some of that with with the audience. So but I'd love for you to start. On your website, you call yourself, you say, I aspire to be a quantum chemist in disguise in the department of mathematics, which I think is very intriguing.
Sebastian Hassinger:Introduce yourself if you don't mind, and and give a little bit of your story of of how you how you ended up being an aspiring quantum chemist.
Lin Lin:Absolutely. Yeah. Those is, also very much related to why I got into quantum computation and, for this podcast. But I think indeed, some mathematician, my path is a little bit unusual . So I'd like to share a little bit Please.
Lin Lin:With the audience. So, I majored in computational mathematics in Peking University. So part of the reason was I was, intrigued by the possibility of modeling nature through computation. Mhmm. So doesn't sound like too far away from quantum computation, isn't it?
Lin Lin:So I took a course from physics department on quantum physics. I was very intrigued by the bizarre physics and possibilities, in those equations. And then one day I read a book that's related to a topic called a first principle based modeling. Mhmm. Let me explain a little bit.
Lin Lin:So first principle means minimal information.
Sebastian Hassinger:Right.
Lin Lin:That's just something just sounds so cool Yeah. And very much attractive to mathematical oriented
Sebastian Hassinger:Right.
Lin Lin:Right. Person like student like me. So, what it means is that you would like to model a chemical system, for example, with a minimal amount of information. Right. That are the atomic species, which means what types of the atoms are.
Sebastian Hassinger:Right.
Lin Lin:And atomic positions, which means where the atoms are.
Sebastian Hassinger:Right.
Lin Lin:Really, it's hard to imagine you can model a system with information less than that. Yeah. Right? True. Yeah.
Lin Lin:So so, so this, like, first principle based modeling, what you do is to solve equations, pretty complicated equations. That's not like, you solve the, like, Newton's law, where you have, let's say, one particle, then you can characterize the position of the particle with three numbers and the velocity of the particle with three numbers that's six numbers you have 2 particles I have 12 numbers 3 particles, 18 numbers, so on and so forth. Right? So so this thing, they call it increases linearly.
Sebastian Hassinger:Right. Right.
Lin Lin:But, chemical systems in need to solve not Newton's equation, but Schrodinger's equation. They have a very very distinct feature. So what it does is you have one particle, you solve a 3 dimensional problem, which is fine, but, when you go to 2 particles, you don't solve, like a 6 numbers, you solve a 6 dimensional problem. Right? That's already you uses mathematical construction.
Sebastian Hassinger:Right.
Lin Lin:Goes beyond the three-dimensional or 3 plus one space time that we're in. Right? So it's already uses this linear algebra thing to think about.
Sebastian Hassinger:And that's because of the interaction at the quantum level, the subatomic level between those particles.
Lin Lin:Right. So they really interact with each other in a fundamentally different way from classical particles. So by the time you get to about 10 or so particles, you just literally cannot use the, best supercomputer in the world to store the information of this tiny bit of quantum system. But if you could solve that, as Paul Dirac said nearly a 100 years ago, almost most of the physics and almost the entire chemistry can be solved. Right.
Lin Lin:Just by solving those equations. Right.
Sebastian Hassinger:That's a great quote.
Lin Lin:Right. I mean, it's a really amazing foresight and that pretty much guided the development of computational physics, computational chemistry Right. For the next 100 years. Right. So, I was very much intrigued by this possibility.
Lin Lin:And when it, about, reaches at the time that I need to, go to grad school, I thought I wanna do that. So I was very fortunate to go to Princeton University and work with a mathematician, professor Wienan E. His last name is very special, just a single letter e. Oh, wow. But it pronounces in Chinese as and, also professor Roberto Carr in chemistry.
Lin Lin:And, so Roberto Carr is, I mean, has very Mhmm. Many well known works. One of the most well known ones probably is, together with a long time collaborator Michele Perinello from ETH Zurich. They proposed in 1985, that's the year when I was born, and, this is so called car parallel molecular dynamics. It was a big deal.
Lin Lin:So for many years, actually decades, carparanellomolecular dynamics was almost synonymous to first principle molecular dynamics. It's a big deal. Mhmm. So, so I wanted to, like learn more, like work on this direction. In particular, one of the projects I worked on is, to, work on better algorithms for one type of first principle theory called the density function.
Sebastian Hassinger:Right. Right.
Lin Lin:So density functional theory, roughly speaking, is some sort of like a bizarre field, or like a theory that says, I said if you have a 100 particles then your principle solving a 300 dimensional mind boggling Right. Like high dimensional problem. Density functional theory says no, you just solve a three-dimensional problem. Mhmm. But you need to make some approximations Right.
Lin Lin:And so on and so forth. Right. And in the past few decades, people found that about 95% of the time, I mean, I'm quoting some, one from chemist, that this is just good enough. Right. Okay?
Lin Lin:The rest of the 5% is really hard. Right. We're gonna come back to what's this connection with the quantum computation.
Sebastian Hassinger:So so just to make it connected, so Perdello Carr, how does that, related to DFT?
Lin Lin:Yeah. So a great question. So the Carr Perinello molecular dynamics is really a way to combine DFT, which is a quantum theory, with Newton's law of motion, which is classical physics that allows atoms to move.
Sebastian Hassinger:Right. Okay.
Lin Lin:So I also want to say that because this DFT, density functional theory, is so useful that its inventor, professor, the late professor Walter Kohn received the Nobel prize in 1998.
Sebastian Hassinger:So
Lin Lin:it is a really really successful theory. But, although you're solving a three-dimensional problem, which means you can do that with a regular computer, it's still pretty slow. You can solve it with a few tens of atoms, hundreds of atoms, but by the time you reach about 1,000 atoms, 10000 atoms, this thing becomes very slow. Mhmm. And, sometimes even impossible to do this kind of calculations.
Sebastian Hassinger:Right.
Lin Lin:So what we thought is whether there's a way to really radically reformulate the problem so that you can do this large scale calculation faster. Okay? That's one of the main projects I worked on during my PhD.
Sebastian Hassinger:Right.
Lin Lin:So I was very fortunate to work with a group of really excellent, researchers, including by then the grad student, Jianfeng Lu. I just heard like, half an hour ago that, he now is the James Duke Distinguished University Professor in Duke University. Nice. So Professor Lexington You knew him when? Yeah.
Lin Lin:When we were both grad students together. Yeah. So so and, so, there's, Lexin Ng, who's now professor in the math department of Stanford, and Doctor. Chao Yang who's a senior, research scientist at Lawrence Berkeley National Laboratory, who is also later my postdoc mentor when I was a ARRAS Fellow in Lawrence Berkeley National Laboratory. And also a group of other amazing collaborators.
Lin Lin:I was very fortunate to work with them and we radically reformulated how you solve density functional theory. It's really different mathematical formulation that allows you, you know what, finally to do this 10,000 atom, even 100,000 atom Right. Calculations on supercomputers. Right. And those things eventually got into community electronic structure software packages.
Lin Lin:So I was later in 2014 hired, as a faculty member by the Department of Mathematics at UC Berkeley. I think partly because of that work. And,
Sebastian Hassinger:I imagine so. And,
Lin Lin:I then continue working on different aspects of density functional theory. I don't want to get too much into that. And, then came to 2018, which is very much a watershed a year for me. Mhmm. It was a few reasons.
Lin Lin:One is, I was tenured that year. Nice. I realized, well, something has gone right. Right. Exactly.
Lin Lin:So, okay. And then people told me, well, it is time to, be a little bit crazy. Right. I thought okay, let's use my personality pretty well. So I said remember this density functional theory is extremely successful over 95% of the time.
Sebastian Hassinger:Right. Right.
Lin Lin:But the rest of the 5%, people are kind of a little bit clueless. I mean, there are a lot of different theories, but there's no perfect thing. Is there
Sebastian Hassinger:is there any I mean, is there any way to generalize that 5%? Is there something, some attribute or some, is there something that makes them more difficult?
Lin Lin:They are. So, parts of the, like a reason that people often talk about is that the remaining roughly 5%, a lot of them are so called strongly correlated, which means the rest are 95% are weakly correlated.
Sebastian Hassinger:Right.
Lin Lin:But it's kind of So the approximations
Sebastian Hassinger:do an okay job with a weak weak.
Lin Lin:Right. But also what is weak and what is strong is constantly being redefined, but that's like just like a loose term saying it is pretty difficult. Right. Okay? And, it is rather hard for you to characterize this strong correlation just using the three-dimensional electron density.
Lin Lin:You just wrong out the information a little bit. Right. So, so I thought like okay, to be a little bit crazy you wanna go a bit big and bold. What can be crazier than trying to solve the remaining 5%.
Sebastian Hassinger:Yeah.
Lin Lin:That means you kind of need to go beyond the density functional theory, and try to solve the original 303,000,300,000 dimensional problem whatever that is. Then around 2018 there are 2 big things happening in science. Of course, they happen they happen in a continuous fashion. One is AI, right? A lot of people might have heard that.
Lin Lin:And the other is quantum. Yeah. So both share the same, they are very very different topics to start with, but both share the same trait in the sense that they're almost designed to handle this high dimensional problem. Let me start with AI. Right?
Lin Lin:So because AI is, let's say, like speech recognition, image recognition, chat gpt, those are incredibly high dimensional problems.
Sebastian Hassinger:Right.
Lin Lin:And the AI just solved the problem like that. Well, it took many years. A lot of up and downs.
Sebastian Hassinger:A lot of GPUs.
Lin Lin:A lot of GPUs. But but but finally they solved that. Yeah. And, it turns out AI could also be very helpful for solving the high dimensional problems in quantum chemistry as well, and people are working very hard on that.
Sebastian Hassinger:Right.
Lin Lin:The other is quantum computing. That is Feynman's original vision for doing that all the time. Yeah, I mean, quote, ubiquitous quote, right? I mean, and, it was, he was really, like a visionary, and, yeah, it's incredible foresight. Whenever you go back and see those, and people like Feynman, like Von Neumann, you're really amazed by like how far they could see, right?
Sebastian Hassinger:It is with the benefit of hindsight. Right? I mean, it is with the benefit of hindsight. I realize, like, I think about this all the time, and in 20, 30, 40, 50 years Yeah. Who are we gonna be looking back to and going like, you know what?
Sebastian Hassinger:Lyn had it exactly right
Lin Lin:or whatever. No. But I mean, we don't know yet. We don't know. Yeah.
Sebastian Hassinger:Yeah. It's like almost survivorship bias of the idea.
Lin Lin:Right. Right.
Sebastian Hassinger:The idea maintains relevance and gets even more relevant and becomes more and more prescient over time? Because right now, we don't know.
Lin Lin:We don't know. Right now, we don't know. But Fireman didn't just come up with a wild No. No. No.
Sebastian Hassinger:He's got a good track record.
Lin Lin:Yeah. Neither did John Von Neumann. No. Right? Yeah.
Lin Lin:So It wasn't luck, for sure. It doesn't seem to be luck. So, so along this AI thing, and, so so well, let's just go big and let's look at both. Mhmm. So both are like a bullet trains.
Lin Lin:Yeah. Right? And so how to hop on 2 bullet trains at the same time.
Sebastian Hassinger:One foot on each.
Lin Lin:One foot on each. And, hopefully, you're still alive by the end of the trip. So and and hopefully they're going along the same direction. Yeah. Exactly.
Lin Lin:Exactly. Yeah. So that's what they did.
Sebastian Hassinger:I I wanna just for a second. So is the reason that they're both good at high dimensionality because at their core, their approach is to to matrix matrix calculations.
Lin Lin:I think there are really fundamental different ways of looking
Sebastian Hassinger:at the high dimensional things. But they are looking at matrices, like, in very fundamental different ways. But is that does that is is that why they're useful in high dimensional?
Lin Lin:I think that's a very deep question. I think the probably the real answer is we don't still don't have a great explanation.
Sebastian Hassinger:Okay.
Lin Lin:But at least on the surface, why a very complex high dimensional objects
Sebastian Hassinger:Okay.
Lin Lin:That is goes way beyond the linear regime that people used to work with. Right? In neural networks, the whole idea is very simple nonlinear functions can represent very complicated and even universal objects. Yeah. Which is quite amazing, right?
Lin Lin:Yeah, it
Sebastian Hassinger:really is.
Lin Lin:Quantum is the opposite.
Sebastian Hassinger:Right, okay.
Lin Lin:So, quantum It's so interesting. Quantum is the opposite. So, quantum, way is to almost use the brute force
Sebastian Hassinger:Right.
Lin Lin:To represent the high dimensional thing, and using
Sebastian Hassinger:linear stuff.
Lin Lin:Right? So really they're both similar to each other, but also polar to each
Kevin Rowney:other as well.
Sebastian Hassinger:Right. Right.
Lin Lin:So so I I find this is really interesting, that's why I say, I mean, we hopped on 2 bullet trains and hopefully they are moving along the same direction, and we started to learn and then COVID hit.
Sebastian Hassinger:Right.
Lin Lin:That didn't quite help either, but, amazingly I worked really worked with amazing collaborators and the group members. Just want to mention in passing that along the AI side that, so, my former advisors, Wenon Er, Roberto Carr, with their superstar student back then who's now a CEO and of, like, a company called the deep, deep, deep tech, I think. Yeah. So like a tech company on this so called deep molecular dynamics. So they came up with really efficient ways for parameterizing the so called interatomic potential.
Lin Lin:Mhmm. That means you're really trying to solve the chemistry problem, bypass all this entire electron structure steps, and try to come up with something that is useful for chemistry. Wow. So, they came up with a method that's in physical review letters in 2018. And in 2020, so they thought, we wanna, like, scale this up.
Lin Lin:And how to do that? Turns out we had a former group member, professor, like Weiler Jia from our group, who is now a professor in University of Chinese Academy of Sciences. And he's really, really good at scaling things up. So finally, he scaled things up from a calculation around, let's say, 10,000 atoms or so, which is still pretty good, to a 100000000 atoms simulation molecular dynamics with the first principle accuracy. Wow.
Lin Lin:So that year, that work won the Gordon Bell prize. So in 2020, and I was very proud to be on the team, although I did quite little and it's really their work. And, so the Gordon Bell prize, in case you don't know, you can go on to the Wikipedia, and there's an interesting quote saying that's like the Nobel Prize in supercomputing. I think it's a little bit over. Maybe.
Lin Lin:Yeah. But, yeah. But, but I I think that's a really
Sebastian Hassinger:I mean, Gordon Bell, founder of Intel, so
Lin Lin:I am. Important person. Important person, and I think it's a really amazing teamwork.
Sebastian Hassinger:Yeah.
Lin Lin:But then my interest, I mean that was great on AI side, but AI it creates this sort of inter atomic potential, you still need some training data, isn't it? So this training data requires still requires you solving that remaining 5% of the problem. So it doesn't really nail the problem. Right. It may once you can solve those 5%, AI tells you how much more you can do Right.
Lin Lin:With those. I mean, it's just amazing things you can do. But you still need to find the good training data.
Sebastian Hassinger:Right.
Lin Lin:And quantum computer is almost one of the best things that, humankind, right, has come up with and that could potentially solve that problem. Right. So I was deeply intrigued, it's a long introduction before my quantum journal Fascinating. Journey, and, yeah. So so so now it's about, like, talking about, like, quantum algorithms.
Lin Lin:Right. Right. Kind of quantum computation.
Sebastian Hassinger:Yeah. Yeah. So, okay. So if I'm following then, what you're saying is that this incredible AI algorithm for extrapolating from the training data up to 100000000 atoms needs that high fidelity simulation to start with the solution to that falls into that 5% of highly correlated systems. Yep.
Sebastian Hassinger:And that's exactly what a quantum algorithm running on a quantum computer can provide. So it's almost like the seed of the training data that'll get you to that 100,000,000 atom scale.
Lin Lin:Hopefully so.
Sebastian Hassinger:Hopefully.
Lin Lin:And, actually, that's if this, like a program works, that's probably one of the best ways to use a quantum computer. Because really I want to say that the more, the less you know about quantum computer, the more you think you can, it can do. The more you know it, you realize the limitation. Okay? True.
Lin Lin:So so, I mean, if you don't know much, I think, oh, wow, we have this eventually, we'll have this working quantum computer. Let's throw away our laptops Right,
Sebastian Hassinger:right, right.
Lin Lin:Replaced by a quantum computer, it's gonna process my database faster. What was your Apple watch? Yeah, maybe I'm gonna do, play quantum games. By the way, there are. There are.
Lin Lin:Yeah. Running on classical computers. You work a little bit different way from how you think about it, or in these Netflix movies. Right? And, yeah, this quantum I mean, it's just so powerful that you have to destroy it in order to save the world.
Lin Lin:Doesn't quite exactly. Not quite. No, not quite. So right, so the more you know, the more, you also know about its limitation. Right.
Lin Lin:So for chemistry, I really think that one of the best use cases, if this whole thing work at all, is that you give some go back to the first principle modeling. You give the atomic species what types of the atoms are.
Sebastian Hassinger:Right.
Lin Lin:Give the atomic positions where the atoms are. You, which is a quantum computer, spells out the energy of the system.
Sebastian Hassinger:So ground state, excited state?
Lin Lin:It could be ground state, could be excited state. The ground state, by the way, is the lowest energy of the system. That's already very useful. I think it is, maybe a little bit useful to explain why we we care about grounded energy. Right?
Lin Lin:So so why ground state energy? This is actually a very interesting question, and the answer is not that obvious. So in chemistry we care about the ground state energy which is just the lowest energy level of this so called hamiltonian. Okay? It's only one of the numerous energy levels Right.
Lin Lin:Of the system. Why do we care about this one? Furthermore, if you know a little bit about statistical mechanics, you know that at the room temperature, all the energies, they should be weighted. Right. Right?
Lin Lin:By the temperature called the Boltzmann scale, like, factor.
Sebastian Hassinger:Right.
Lin Lin:So not only the ground state energy, but also first excited, second excited, tense excited. Right. This should all play some effect. Why do chemists, and not only chemists, but also material scientists, they care about this ground state energy that presumably should only be dominated at absolutely zero temperature, and we're not living in 0 temperature. Right?
Lin Lin:Thankfully. Otherwise, things are moving really slowly, and it's hard to imagine how life forms and so on and so forth. So the reason is because of the gaps between the energy levels in a typical chemical system. So the gap between a typical typical chemical system, also in materials, is on the order of electron volt. It's kind of like a weird unit of, energy.
Lin Lin:So let me just convert that to temperature. Which means an electron volt is roughly, if you convert to the energy in the unit of temperature, is about 10,000 Kelvin or 10,000 Celsius at that time. At that level, 273 doesn't quite matter, so let's call it 10,000. So Close enough. Right.
Lin Lin:And we live, by the way, around 300 ks, right? So which is very very tiny compared to the typical energy gap of the system. I say typical because I'm not talking about things like bizarre stuff like superconducting where the gap is like a few Kelvin. That's why it needs to be really cool. That's where the quantum computer needs to be stored in this freezer.
Lin Lin:Right?
Sebastian Hassinger:So And just so Hamiltonian is is the dynamic of the of the the chemical system. When you say chemical system, that term, does that refer to, you know, a chemical? Like, let's say, I don't know, acetaminophen or something, an aspirin, like, or is it a chemical reaction, like 2 substances combining?
Lin Lin:That's a great question. Yeah. So what is, yeah. So, let's say it is, I'm more, talking about, let's say, aspirin, right, sitting there. Okay.
Lin Lin:So the chemical reaction is actually extremely closely related to those ground state energy combination. So what is a chemical reaction? A chemical reaction is not that much more than atoms, the atomic configuration, they're changing a little
Sebastian Hassinger:bit. Right.
Lin Lin:But why do, molecules and atoms they want to stay in one position rather than others? It's because in some configurations the energies are lower so they are more favorable and, in some positions the energies are higher so they are less favorable.
Sebastian Hassinger:So because the electron volt gap is so high.
Lin Lin:Right. The typical separation between this kind of, like energy levels is on the order of EVs. I'm not talking about everything but it's typical. It is on the order of 1,000 to 10,000 kelvin range. So the 300 room temperature becomes quite small compared
Sebastian Hassinger:to that.
Lin Lin:So so that's why in chemistry and material Right. So once you so called Boersman weighted, which is you weigh the importance of energy levels by this by this relevance factor, So the ground state becomes extremely good approximation to I see. How the molecules and the materials behave.
Sebastian Hassinger:Right.
Lin Lin:Right? So that is why although we're living in room temperature Right. We care about this zero temperature Right. Property. Right.
Lin Lin:So what you said I think is a really important question and, that is, I mean, really governing why the scientists are working on this kind of thing. Right. So, go back to the this, like ground state, like energy thing. So you're you do this first principle based modeling, You're given atomic species, atomic position, you wanna compute the ground state energy using quantum computer, for example. You do this for different types of atomic species and different atomic positions, and you collect a lot of training data.
Lin Lin:Mhmm. I strongly believe once you have those training data, AI could take over from there. Right. Right. And they're gonna do amazing things.
Lin Lin:I think they're really gonna scale up and do amazing, amazing stuff.
Sebastian Hassinger:That's so cool.
Lin Lin:So I think letting AI and, quantum computers, they're really not competitors to each other Right. But more like in the future Complimentary. They should complement each other.
Sebastian Hassinger:Yeah. That's so interesting. And I mean, another topic that we were talking about, which I think this feeds directly into, I said at some point that there's in the outside of the field itself Yeah. There's something of a perception, a growing perception of, you know, there was Shor's algorithm in 1995. It's a very easily understandable sort of thing that quantum computers can do that classical computers cannot do, can factor large numbers to their primes.
Sebastian Hassinger:And there haven't been a lot of notable, sort of, very clear examples of what quantum computers will be able to do since then. I mean, there have been some advances, but there's, I think, a general perception that maybe things are not going as quickly as we'd hoped, or, you know, that progress is slowing. And it sounds to me like, and you've said this to me before, so I'm basing this on what what I'm hearing from you. But but what you're describing is is actually incredible progress in a in an area that maybe is harder for nonscientists, nonresearchers to understand. It's it's I mean, what you're describing is like revolutionary breakthrough in terms of our ability to understand quantum chemistry, and therefore, you know, quantum reaction or chemical reactions, chemical properties, all those types of things.
Lin Lin:Yeah. This is really a fascinating question. I'm really glad that we first had the Zoom call. Yeah. Because, really when you mentioned a version of this question the first time I remember I froze for 2 cast seconds because I was like, what?
Lin Lin:And I could, from my perspective, amazing progress has happened just in the past 10 years. And you're saying there's no progress since the short? How about Not to be. Yeah. Yeah.
Lin Lin:No. No. Yes. Yeah. Or perception.
Lin Lin:Right. Right.
Sebastian Hassinger:But then many people are
Lin Lin:saying, many people are saying, but then I realized that, I mean, I'm not the first one on this podcast. You have interviewed amazing people like Scott Aronson, Doreida Ahonnovel, John Preskill, right, right. Stephen Girvin. I mean, how come this question arose after this, like, I mean dozens or several dozens. That's my perception.
Lin Lin:Right. Right. I I It's a thing that I think,
Sebastian Hassinger:I mean, what you just walked through in terms of even the ground state and and the explanation of that model of quantum computer being able to provide training data for an AI model that can scale up to a 100,000,000 atoms, that puts it in terms that that I think are very concrete that, that will, exactly, you know, be a counterargument to the the lack of progress. I mean, that's clearly progress, right?
Lin Lin:Yeah, there's clearly progress and then I went back and dwelled on it and realized there's actually might be some deep reason. At first, I thought you were being deliberately provocative. A little bit. A little bit. Yeah.
Lin Lin:To see my reaction. My reaction was I froze, and well, glad I'm not freezing right now. But I realized there's really something deep behind your question, so let me think about it like this, right? So Shor's algorithm, in 1995, alright, 30 years ago, was something that is truly spectacular. That is something that is very rare, to see even in science.
Lin Lin:I mean, he had this amazing run
Sebastian Hassinger:Yeah.
Lin Lin:That first developed Shor's factorization. By the way, let me just say again, Shor's algorithm is to factorize a big integer, right? Number into the product of 2 prime numbers. This thing has amazing, a very very important applications Right. In cryptography, why we believe our bank accounts are safe, so on and so forth.
Lin Lin:Right. And, the reason why we believe it's safe is because classically it's really hard.
Sebastian Hassinger:Fast, very hard.
Lin Lin:Yeah. And the short showed that once you have a perfect quantum computer, it becomes quantumly easy. Yeah. And furthermore, the it is not only easy in the abstract sense, it is in the extremely concrete sense. The inputs of the problem is a single integer which is understandable by human beings and by classical computers.
Lin Lin:Yeah. The outputs are 2 integers. Yeah. We're trying to That's our 2 prime numbers, which is also understandable by human beings. Basically, yeah.
Lin Lin:Right. Right. Yeah. So so, so the the the the prime numbers both understood, standable by human beings and the classical computers. So very clean input, very clean output, very low quantum cost, and very difficult classical.
Lin Lin:I mean, it is just a almost watertight argument that has closed all the possibilities that, you can say, hey, there's no quantum speed up. Right? Right. And, not only that, and he kept, saying people say, hey, quantum computers may make errors. I'm gonna develop error correction.
Lin Lin:And,
Sebastian Hassinger:less than a year later.
Lin Lin:Yeah, less than a year. And people say, okay, that's pretty good. But, you know, the error correction may itself make some error. Peter Shor came back and say, I'm gonna do it the full tolerance. So I Yeah.
Lin Lin:I know. This type of amazing runs just happen very rarely. Yes. In, like in not only in quantum physics, but also in science of law.
Sebastian Hassinger:Yeah. And everything.
Lin Lin:Yeah. Yeah. Kudos to Peter Shorin. I'm sure many other, like, speakers have talked about this over and over, but I don't think it hurts to reemphasize, just to talk about this, that tagger story. A little bit unfortunate thing.
Lin Lin:For everybody else. For everybody else. Is that I mean, why you could come up with such a perception is that in the next 30 years, right, we're gonna, I mean 2025 is coming. Yeah. And, not a single application or algorithm reached the level
Sebastian Hassinger:That's right.
Lin Lin:Of Shor's algorithm.
Sebastian Hassinger:That's right.
Lin Lin:Let me repeat what I said, what Shor achieved. Yeah. If you think about it, it's remarkable. Yeah. So the key thing to achieve quantum vantage by the way, quantum vantage is a term that says quantum computer is doing something that is useful, 1.
Lin Lin:Mhmm. And 2 is that it is better than classical computers on a certain task. So it means that the task needs to be quantumly easy and classically hard. Mhmm. Okay.
Lin Lin:So how to do that? You, so I gave a talk yesterday, and it's a very simple message. There's no mystery behind it, and very easy to honest with as well. Easy means that the input to the quantum computer used to be easy, the cost should be low. The output of the quantum computer should have low cost, which means that you shouldn't just measure this, quantum computer like, for like, let the quantum computer output like a trillions of numbers.
Lin Lin:Right. The quantum computer is really really bad at doing that. Yeah. It's very good at something, but it's very very bad at that. Yes.
Lin Lin:And, the quantum running cost, which means the circuit depth cannot be too high.
Sebastian Hassinger:Right.
Lin Lin:And it also needs to be a classically hard problem. Right. Can you say that there's no progress satisfying all these criteria, for, like after short? No. Actually there are a few.
Lin Lin:Right. But very unfortunately, those problems are a little bit contrived Right. Compared to shortest factorization. Maybe to most of the people, cryptography is pretty contrived. But it has such utility as It's such utility.
Lin Lin:Right? Right. So so I may not I may not be thinking about factorizing big numbers all the time. No. But I wanna log on to my bank account from time to time and make sure that money isn't going somewhere that I don't know, right?
Lin Lin:And, but the other progress have to say they're unfortunately more contrived than that. Mhmm. Which is a very important reason that the public isn't quite aware. But also you can talk about this quantum advantage, quantum utility for things that you do care about, let's say the ground state energy of, like a first principle based model. Right.
Lin Lin:Right? But the argument is not as tight. So it could be, reasons are, there are various ways. Maybe the input problem isn't very easy to solve. You need to provide a quantum computer with very complicated input state in order to start solving that problem.
Lin Lin:Right. Maybe your quantum algorithm needs to, like, require the quantum computer to output tons of tons of tons of tons of information. Right. And as I said, quantum computers are really bad at doing that. Maybe the quantum algorithm is not running as fast, but then that part turns out to be one of the easier problems to address.
Lin Lin:Right.
Sebastian Hassinger:Or Just increase n to the point where the classical run time is way higher.
Lin Lin:Or you're talking about a problem that's not for quantum chemistry, but maybe for some other problems that classical is hard but not that hard.
Sebastian Hassinger:Right.
Lin Lin:Right? So classical is not like a super hard which also makes it hard to argue there's a significant quantum speed up. Yeah. There's also something I didn't talk about, which is you also don't want to touch the problems which is classically hard and the quantumly hard. Right.
Lin Lin:Right? Quantumly easy, classical hard
Sebastian Hassinger:Right.
Lin Lin:Sounds very easy to do, but once you scrutinize, examine all those aspects, you realize that things are not so easy.
Sebastian Hassinger:Right. Right.
Lin Lin:So indeed there is really good. I mean, go back as a long answer to the, short question you raised earlier that says, is there progress in quantum algorithm in the past 30 years? I would say definitely yes. In fact, in the past 10 years, which I can elaborate a little bit later, that, there is a tremendous progress that has been made in quantum algorithm that I I really have really really strong reason to believe those are some of those algorithms are the ones that will be executed when we have better hardware.
Sebastian Hassinger:Right. Right.
Lin Lin:Because they are just so natural and sounds like you're doing the right thing. Right. Those, algorithm were not available, let's say, more than 10 years ago. However, none of them has reached to the stage of a short that claim. But I also wanna say, I mean, for example, in for this podcast, a lot of the audience there, have some level of technical background.
Lin Lin:Right? And it is important to know that, the algorithm and development that happens continuously is not like either you have some groundbreaking or nothing happening. Right? Right. So I think because the community has made such a big progress in the past decade or so or 2 decades, it is very much worthwhile to communicate what really have been achieved.
Sebastian Hassinger:Yeah. Yeah, Absolutely. Absolutely. I'm mindful of time.
Lin Lin:Yeah. But this is what
Sebastian Hassinger:I said before we started recording. It's probably gonna end up being a multipart conversation. I mean, I'm happy to. So many incredibly interesting detours. But, so, I mean, to bring it back to the quantum chemistry, it does feel like, like cryptography, it's a, you know, that one solving that one problem is going to have incredibly broad implications.
Lin Lin:I think that's gonna have enormous impact in the society. Yeah. So I mean, one thing is drug discovery, right? Yeah. So but, I don't wanna, like use the word drug discovery to just to say that quantum computer is going to solve all the drug discovery problem.
Lin Lin:Once you have a quantum computer, we're gonna have better drugs. I mean, it's a very complicated technical progress. But what quantum computer can do, and I think it's a very promising way of, doing things, is that quantum computer allows you to, compute very accurately the energies of certain very challenging atomic configurations. Those are invaluable training data. Right.
Lin Lin:For example, for your AI, for some other things, you integrate this into the workflow of other things. Right. Finally, that allows you to model the, the drug database Right. Or chemical database
Sebastian Hassinger:Right.
Lin Lin:Much more accurately, allows you to do better screening, better discovery, and I think that's really a very promising path down the line.
Sebastian Hassinger:Yeah, absolutely. And then of course, anything that's applicable to chemistry is also applicable to material size. So material size,
Lin Lin:I think, the materials g norm, project might have a huge boost because you just just buy the fact that you have all this new highly accurate training data that Right. People can start mining those kind of database. Right. And, you know, I mean it's, it's quite, imaginable that people will get something really, really useful out of those databases. Absolutely.
Sebastian Hassinger:Okay. I think I'm gonna put a pin in the conversation right there. Short.
Lin Lin:Yeah. Yeah.
Sebastian Hassinger:Because we've talked for 30 minutes, and that's a good amount of time to blow people's minds. But this has been amazing. Thank you very much for joining me, and I'm definitely gonna hold you to the the the offer of a part 2 Oh. And maybe subsequently part 3 and part 4. So Thank
Lin Lin:you very much, Sebastian. It's really a privilege to be here.
Sebastian Hassinger:Oh, it's my privilege. Thank you very much.
Lin Lin:Thank you very much.
Kevin Rowney:Okay. That's it for this episode of The New Quantum Era, a podcast by Sebastian Hassinger and Kevin Roney. Our cool theme music was composed and played by Omar Khosstah Hamido. Production work is done by our wonderful team over at Podfi. If you are at all like us and enjoy this rich, deep, and interesting topic, please subscribe to our podcast on whichever platform you may stream from.
Kevin Rowney:And even consider, if you like what you've heard today, reviewing us on iTunes and or mentioning us on your preferred social media platforms. We're just trying to get the word out on this fascinating topic and would really appreciate your help spreading the word and building community. Thank you so much for your time.
Creators and Guests
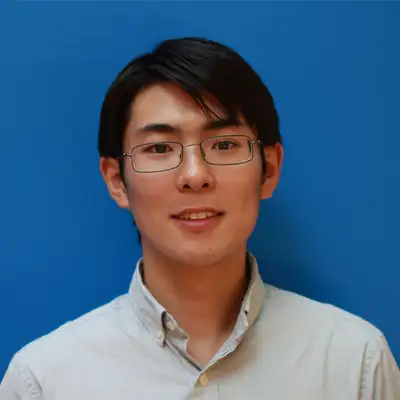
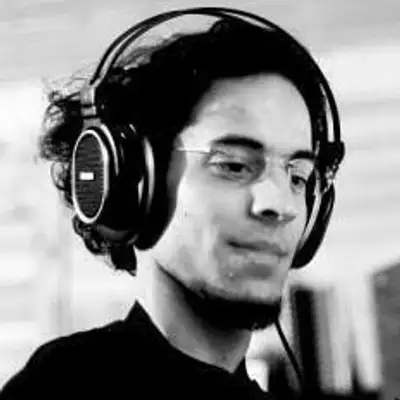
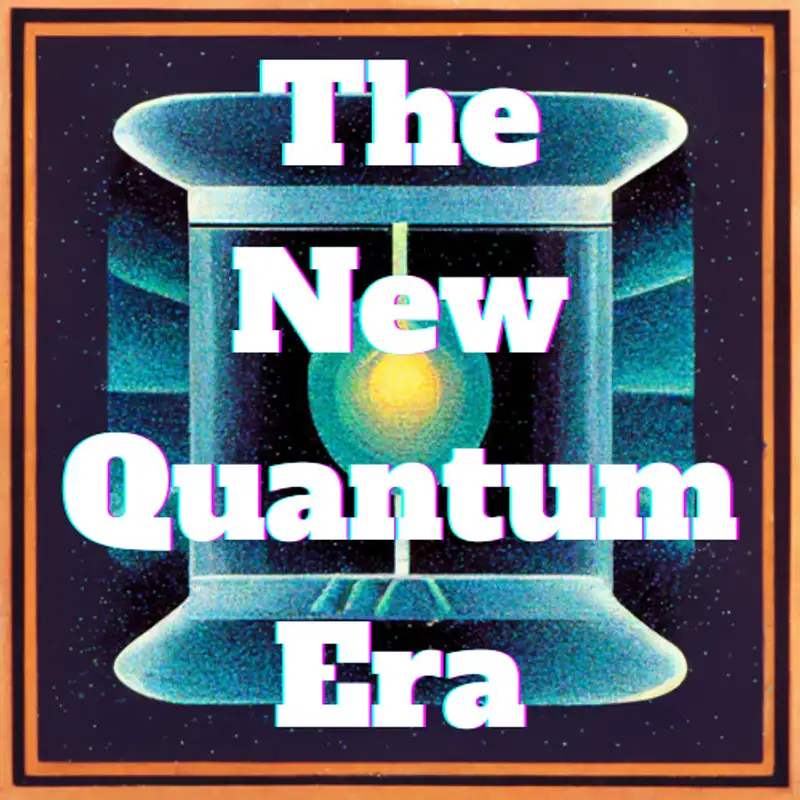