Adiabatic and Counterdiabatic Quantum Computing with Dr. Ieva Čepaitė
Download MP3[00:00:00] Kevin Rowney: Welcome back. today we have a fascinating interview with Dr. Ieva Čepaitė, and she's a physicist working on quantum computing at a startup called. Phasecraft in Bristol, England. She worked primarily on what's called, counterdiabatic methods that help optimize the control of many body quantum states.
[00:00:38] there's, actually quite a lot of interesting quantum computing, algorithms out there. Many people know, of course, of Shor's algorithm, but there's actually quite a, quite a bit bigger toy box, of different options here. So we're gonna get some interesting insights. from, from,
[00:00:53] Dr. Čepaitė today. I feel confident, that she's going to give us a nice overview of the landscape of some of the new algorithms, now available within the industry. Sure.
[00:01:03] Sebastian Hassinger: Yeah, Kevin, I'm quite excited too. Ieva is a recent PhD recipient from Strathclyde. as you said, she's joined, Phasecraft, which is a really interesting startup in the UK, with some very talented researchers.
[00:01:16] and the work that she's done as Part of her academic career is also very interesting. I encountered Ieva, I think on social media first. she's quite a good science communicator, so I'm very much looking forward to this conversation.
[00:02:04] Welcome back. Thanks for joining us. We've got a special guest with us today. A quantum researcher based in the UK, Ieva Čepaitė. she's a quantum, algorithm researcher, recent PhD, recipient and, recent employee at Phasecraft, a really, Interesting and powerful startup in the UK quantum space.
[00:02:26] so welcome to the show, Ieva. Thanks for joining us.
Ieva Čepaitė: Thank you for having me
[00:02:30] Kevin Rowney: we're doing, Ieva, often we begin the, these interviews with asking, people how, what was their route towards coming into quantum computing? it's usually a, an interesting kickoff and there's usually some fun nuance to that story.
[00:02:44] Could you give us a little background as to what led you to, to this outcome?
[00:02:49] Ieva Čepaitė: Yeah, it's not the first time, it's not the first time I've been asked. the truth is, when I was an undergrad, so I was studying in my undergraduate physics degree, physics and computer science combined in Edinburgh in Scotland.
[00:03:04] And at some point, for whatever reason, I noticed some news article, something on quantum information. And I think at the time, I just started learning about quantum mechanics. And so it piqued my interest. And I started reading a bit about it and I was like, okay, this sounds really cool. I want to know more.
[00:03:22] and I was very proactive. So I found out that there was one group at the university working on this and they were the computer science department. So I'm not in the physics field where I was at the time, as strongly, but I, so this was, run by professor Elham Kashefi. who works broadly on, verification, quantum cryptography, and quantum machine learning, and I think there are many, different things.
[00:03:47] So I started emailing her over and over again, I think for several months, because she's obviously very busy, many people are in her position. Until one day she actually replied, and she said, Oh, yeah, sure, you can come by and sit in on our group meetings. If you're, that desperate, uh, and and I did, and I obviously didn't understand anything they were talking about at the time, but I thought it was really cool and the people were really nice, and I ended up deciding to do my bachelor's thesis, roughly on the topic, and then later on my master's thesis, and by that point, I'd I've fallen deeper and deeper into the hole because I was like, this is really cool.
[00:04:25] There's so
[00:04:25] Kevin Rowney: many caught in the spell of quantum.
[00:04:27] Ieva Čepaitė: Yeah, exactly. Yeah. It's a really fascinating field from almost every perspective, right? From the physics side, computer science side, from the philosophy side,
[00:04:37] Kevin Rowney: mathematics. yeah, it's just, it's a, pile of beauty. It really is. Yeah.
[00:04:41] Ieva Čepaitė: Yeah. And so at that point I was like, okay, obviously I'm going to stick with this.
[00:04:45] And, the, only decision I'd really made by the time I, Fin, I'd be done with my PhD was to explore most aspects of it, at least a little bit, which I managed to do actually roughly. like the experimental side, a little bit, the, condensed matter, many body physics, the computer science, the, even the full tolerance for a bit.
[00:05:06] Cause I spent an internship at River lane. So it's, and it's a cool, fascinating field. Yeah. Yeah.
[00:05:12] Sebastian Hassinger: That's great that you have such broad experience. that's one of the characteristics I think that can make quantum computing sort of daunting is, how many different disciplines are coming together to form this new field.
[00:05:25] So what sort of having that broad Is there, does that sort of shape your sort of, sense of where, the, biggest gaps are, the biggest opportunities for progress are?
[00:05:38] Ieva Čepaitė: Yeah, I think that's probably the one thing I feel more strongly that I have a good experience, not that I have a good sense.
[00:05:46] Maybe that's a better way of expressing it. because there is an issue with jumping around a lot, which is I didn't actually dive too deep into any one topic. And that does, I do struggle sometimes with people who are experts, genuinely experts in a particular aspect. But at the same time, I feel like very often...
[00:06:04] If I go to a highly theoretical conference, I'm usually sitting back going, I don't know if the experimentalists will agree with most of the things you're claiming here. And then you go to an experimental conference and I'm like, oh wow, people are really not aware of all the tricks and the ideas that they could use to enhance what they're doing.
[00:06:23] So yeah, that's, quite fun actually.
[00:06:26] Kevin Rowney: The classic polarity of those two subcultures in physics, the experimentalists and the theoreticians, yeah, classic. I gotta tell you, Ieva, it's a great story in terms of, just being a young person, having that ambition to get into this very formidable subject and then keep, keep pushing on...
[00:06:41] Try to make a breakthrough and then be invited into the research lab. that's a, great story of, persistence and getting, the ring. So way to go. it's
[00:06:50] Ieva Čepaitė: also thanks to Elham because I think she's very supportive of these things. She is. not everyone has to do that. And her first response was not.
[00:06:58] Yeah, exactly.
[00:06:58] Kevin Rowney: Props to her too, no doubt.
[00:07:01] Sebastian Hassinger: Yeah, she's great. I think that what they're doing at the Edinburgh School of Informatics is really terrific. They just started that quantum software lab, as part of the National Quantum Computing Center. think that'll be really interesting to see. And then, of course, she's got that, you mentioned, the Edinburgh, Paris virtual quantum lab that she runs.
[00:07:17] So there's the connection with, CNRS in Paris. That's quite, quite fruitful as well. you did, so you did your bachelor's thesis in, I think quantum, homomorphic encryption, right? Yeah, but that,
[00:07:32] Ieva Čepaitė: that honestly, the title looks, fascinatingly scary, but I didn't really do any theoretical work.
[00:07:39] I was mostly getting to grips with the topics. It was mostly learning about... Got it. What quantum... It's just your
[00:07:44] Sebastian Hassinger: bachelor's thesis. That's to be expected. But then, encryption, and then master's thesis was, was more on quantum machine learning. Is that right? Yeah. Yeah.
[00:07:57] Ieva Čepaitė: And
[00:07:57] Sebastian Hassinger: then, and then your...
[00:07:59] And then your PhD is on counter diabetic, phenomena, so that's a very broad range. Is there, out of that range, is there an area that you're focusing in now?
[00:08:13] Ieva Čepaitė: no, in as far as, I don't want to say too much about what I'm doing at Phasecraft, I don't think they mind too much, but I also just don't want to, I'm, not quite sure what I need to talk about, but no, I'm I've enjoyed every single aspect and I think they all at least vaguely end up interplaying on some grand scale.
[00:08:34] Maybe, that's not completely true. Obviously, once you focus in on something, like if I'm doing quantum machine learning, it will be a lot more on the theoretical computer science, like what's, ML if I'm trying to simulate. It's as many people are molecules or something. If I'm trying to do variational stuff, I will probably need a lot more quantum chemistry.
[00:08:51] But at the end of the day, like my PhD was very different to the master's, the undergrad, but all of them come together at some point. You look, get expertise in every field and then you understand how it plays into everything else. So yeah. Yeah. I don't know if that's the answer you were looking for.
[00:09:08] Yeah, no,
[00:09:08] Sebastian Hassinger: no, that's, great. I, that's encouraging to know that even in, in very esoteric topic areas like that, if you, sort, you can find ways to connect them, in a way that, that, that's, that, that is additive, That's,
[00:09:25] Kevin Rowney: impressive. I'm struggling with how best to, if we can, summarize, where, you are with respect to your area of concentration versus the entire.
[00:09:36] landscape of the other algorithms out there said. Is that a, I don't know, fair question?
[00:09:42] Ieva Čepaitė: Yeah, if, just speaking about the PhD, I think you could say this counter diabetic stuff harkens a little bit to quantum algorithm. It's, basically, there's this notion of adiabatic quantum computation or annealing, quantum annealing, D Wave popularized, but I think many people do in slightly different ways.
[00:10:04] This is something that, during my PhD, I focused on. Making the physical experiments more practical rather than a more abstract idea of quantum algorithms. Yes. But it turns out there is a strong interplay between these two because we're at the level where if you want to implement the quantum algorithm on some hardware, you often have to understand the hardware decently well.
[00:10:26] You do,
[00:10:27] Kevin Rowney: right.
[00:10:28] Ieva Čepaitė: This is not as much the case maybe in the superconducting realm and so maybe that's unfair I think the superconducting quantum computers are a lot more hands off Usually people don't talk about the physics as much but I was working with like cold atom stuff Ion traps this kind of thing and there you're writing purpose built almost ways of processing information into it.
[00:10:48] So yeah, I'd say that the stuff I was doing in my PhD at least could be considered a type of algorithm, but it's definitely not what you'd think of when you, talk about an algorithm, even in the quantum sense, because it wasn't very abstracted away from the physics. It was very much what can we do given these very limited operations,
[00:11:10] Kevin Rowney: right?
[00:11:11] I think it might be interesting to our audience to talk about the contrast between, the adiabatic computations and these counterdiabatic systems. and sometimes those are tough questions to ask for a, explain it to me like I'm five rendition of these very sophisticated mathematical concepts.
[00:11:29] But I'm still going to throw that zinger at you and see if we could collaborate on rendering an explanation.
[00:11:36] Ieva Čepaitė: so you want to know what counter diabetic stuff is?
[00:11:39] Kevin Rowney: I think we need to start for our audience by first, giving the overview of what adiabatic is. Yeah. Yeah,
[00:11:45] Ieva Čepaitė: adiabatic computation is basically going, Look, there's some things, some quantum states.
[00:11:53] That we can prepare in a quantum state, it's like a state of a computer, right? It's it's some state of the machine and some of them we can prepare really easily And the way I would usually intuitively explain it is say I have a bunch of magnets and the state I want is all of the magnets pointing up or pointing in one direction I'll just...
[00:12:14] Easy problem to solve that one. Yeah, That's easy because intuitively and for various reasons we know we just Put them in the magnetic field that's pointing in the direction we want them all to point in and this presumably that's where they're gonna go And then we'll get that state right ready to go with quantum systems same thing There's some states we can prepare very easily, we apply some magnetic field some electromagnetic field But then there's some states that we want for whatever reason that are really hard to prepare It's not obvious how to actually get to those states and But we do know that, if we start in an easy state and we slightly vary some parameters, very, slowly, then we should get to the state we want.
[00:12:58] Now, adiabatic quantum computation, the word adiabatic pretty much means you have to do something extremely slowly. If you don't, you run the risk of basically ending up somewhere completely different. and The intuition here is, again, you can imagine with the magnets, if you want to turn the magnetic field, to point in some other direction, if you change the magnetic field super fast, the magnets are going to overshoot for a while and oscillate around a little bit, like this is,
[00:13:27] Kevin Rowney: not even make it to the final destination of the configuration that you're looking for to solve the problem. Yeah.
[00:13:32] Ieva Čepaitė: Yeah. And so this is the intuition between, behind the adiabatic quantum computation which is we want to use this method of doing things extremely slowly to get to some hard state, to something that we couldn't normally prepare.
[00:13:45] now the reason this isn't like a universal solution to most problems because you could say, I could do this for everything, right? Any hard state. They don't want to prepare. I'll just start.
[00:13:54] Kevin Rowney: It solved any problem with almost limitless time complexity on the hierarchy, right? amazing things if, it was easy, but it's not.
[00:14:02] But
[00:14:02] Ieva Čepaitė: it's not, in part because there doesn't always exist a very clean path between some initial state and some final one. but that's not the biggest problem. The biggest problem is that doing things slowly enough. It's usually not possible in the lab. It's just things don't work on that scale, but even if it were easy to do that, you would have what we call decoherence, right?
[00:14:24] Losses. So noise decoherence, these things happen on a much faster timescale. So if you actually try to do this transformation really slowly, you're going to find that there's other. problems that arise in the meantime that are so overwhelming that you end up not achieving anything. So there's
[00:14:38] Kevin Rowney: piles of problems in the way of these adiabatic methods to work in the real world.
[00:14:43] Yes. Exactly. Yeah. Yeah. Interesting. Okay. That's so useful. Thank you. And I, finding, these basic explanations that, appeal to our audience on these technically sophisticated topics. That's, so useful. So I appreciate that. And so having, described adiabatic systems, help us, grapple with this, notion of counter adiabatic systems.
[00:15:05] Ieva Čepaitė: Yeah. the idea is fairly simple. Once you've grasped like, okay, say I've got this thing and I need to do something slowly so that it doesn't overshoot, right? So if I think a nice way to imagine it is if I'm doing something really slowly then at each instant in time It's statically in the most stable position it can possibly be, right?
[00:15:29] So it's if I'm doing if I'm turning my magnetic field really slowly Then almost every millisecond if I look at it, the magnets can be pointing along whatever direction the magnetic field is pointing along, right? so this sort of instantaneous following of whatever it is I'm trying to change.
[00:15:48] Now, if I do something really fast, what ends up happening is obviously it's not following it, so it's out of this ground state, it's out of its most stable state. And what counter diabetic driving tries to do is it goes, what's the force? That's pushing it out of that state, like what's making it, like, how far away is it from that state at each instant in time?
[00:16:11] And can I counteract that? can I just physically go, okay, I know that the magnet is away by this amount of angle, what if I just push it back? And make sure that it stays there, right?
[00:16:23] Kevin Rowney: would it be fair to, characterize this as a, almost an error correction regime, within idiomatic computation? Is that fair?
[00:16:29] Ieva Čepaitė: Yes, it's, like continuous time. So I think of error correction as like discrete things where it's okay, we do discrete steps. We measure, measure, then we correct with counter diabetic things. It's a continuous force that you're applying constantly to keep something vaguely stable, right?
[00:16:49] that's, again, if you implement this counter diabetic drive, if you know what the force is that you need at each instant in time throughout your adiabatic protocol, if you know it exactly, you can perform adiabatic computation infinitely fast. So you can really do it at, any amount of time possible.
[00:17:10] So it goes against the idea of antibiotic. 'cause antibiotic actually says you need to do something infinitely slowly for it to really work. But if you can apply a counter diabetic drive, then you don't really care how fast you do it. 'cause no matter how much you throw the magnet off, off course you can always.
[00:17:26] Presumably corrected
[00:17:27] Kevin Rowney: back. So, interesting. And I'm wondering, there's a whole diversity of different quantum computing architectures out there, right? But I think one of the under acknowledged new entrants in this space is this whole idea of, analog quantum computers that haven't really implemented gates, but they're still looking for magnetic interactions between adjacent subatomic systems.
[00:17:50] And you've got a full icing protocol, so to speak, that's available there. Is it possible that these counter diabetics, algorithms would be applicable to those analog quantum computers? Yeah,
[00:18:02] Ieva Čepaitė: exactly. And that's what our work focused on. Yeah. And I see. Yeah, the, so just to be fair, given, I've given you all the problems with adiabatic with counterdiabetic stuff is that, they're there.
[00:18:18] Yeah. Two fold mainly, but there's more than that, obviously. one is it's extremely hard, if not impossible in many cases, to know what this exact counterdiabatic drive is. in theory, we know this thing exists, but we just can't access it, right?
[00:18:34] Kevin Rowney: Is it then the case that it's just hard to solve for the final equation that defines the error correcting mechanism?
[00:18:40] So there may not even be analytic solutions in some cases. Yeah, so
[00:18:44] Ieva Čepaitė: for small systems, you can work it out. for really small systems, you can get the exact thing and it's really simple because it's related to degrees of freedom, right? It's if I only have three degrees of freedom Then you know, I only need three degrees of freedom to describe this thing yeah, once you get to I don't know 20 qubits.
[00:19:01] That's
[00:19:02] Kevin Rowney: two to the ever present curse of dimensionality. Yes, exactly
[00:19:06] Ieva Čepaitė: So and not only that you need to know that each instance in time and in double quotes So it's not even that you can solve it, you need to know a continuous function that does
[00:19:18] Sebastian Hassinger: So this is where the philosophy comes in, as you were saying.
[00:19:22] Kevin Rowney: yeah.
[00:19:24] Ieva Čepaitė: Everything, we always run into this curse, this is, a thing, no matter what you try to do. so that's, that one issue is, knowing it. another issue is implementing it. So even if you do know it to some degree, these, this force is usually super complicated to the level where, no experimentalist is going to take you seriously.
[00:19:45] You go to them and give them this. You're like, hey, you can drive this thing really fast. You can do this computation, but you just need to be able to implement, this operator or this gate or whatever, and they're going to laugh. Like laughing, because
[00:19:57] Kevin Rowney: it's so insane to try to get it done. it feels like there's a, route here towards possibly some interesting new algorithms for these, analog systems.
[00:20:08] this is thrilling to me to hear because I, I wasn't necessarily, seeing all the way towards the possible commercial, outcomes that might be realizable with analog quantum computing systems, but this seems I don't know, perhaps a pathway towards,
[00:20:22] Sebastian Hassinger: towards that. and I wonder also, it sounds like some of these techniques might help, like a neutral atom array or a trapped ion system with, with achieving greater stability so that you can, you're, when you're applying your gates, you're actually getting a more stable sort of, system overall to, to have longer gate times, et cetera, longer gate coherence, et cetera.
[00:20:43] Yeah. I
[00:20:44] Ieva Čepaitė: mean, this is one of the simpler ways to apply this counter diabetic idea without even thinking about it as an algorithm is to go. Most actual gates that get implemented, like just single operations, that you think of in a quantum computer. They're pulses. They are continuous waves, right?
[00:21:02] That you apply to your system, right? Whether it's an atom or an ion or whatever. And Usually these things are also adiabatic, and so if you want to get better gait, you just apply a counterdiabatic pulse. The nice thing about this is, I told you that for very simple systems, the counterdiabatic pulse is not that hard, so you can actively implement these things.
[00:21:24] You can improve, gaits,
[00:21:26] Kevin Rowney: yeah. That's interesting, so are there already implemented, systems that are making use of these advantages? I think so.
[00:21:34] Ieva Čepaitė: I don't want to lie. I know that, I know people have implemented these things in practice. I think my university, my PhD university, Strathclyde, they have a neutral atom, computer that they're building, and I think they're definitely planning to.
[00:21:51] To implement these things. I should know this because someone from my group has a paper on doing something like this, but I'm not sure to what extent, whether it was implemented or if it's still in the
[00:22:02] Kevin Rowney: works, but Maybe we'll take them on as a guest.
[00:22:03] Yeah.
[00:22:04] Sebastian Hassinger: We'll put the link in the show notes so that people can figure it out for themselves.
[00:22:09] Kevin Rowney: That's really interesting. Just an excellent overview of your work. Thank you so much. I'm wondering, If I'm not mistaken, you're also a specialist in quantum machine learning algorithms. Is that, a fair characterization or did I read your bio wrong?
[00:22:25] Ieva Čepaitė: No, I am by no means an expert. I did, work on it In, I would say, in the early days is, when my thesis came out.
[00:22:34] So at the time I definitely knew probably more than many, partly because not too many people were working on it, I think. but I haven't really worked on it since. And that's, and it's a field that's been accelerating so fast that I, dare and say I, I know too much because I just haven't looked at it in a long time.
[00:22:52] It does
[00:22:52] Kevin Rowney: seem like a fast moving space with a lot of, trial and error on finding, The next new frontier. Yeah, hard to track, to be frank. Yeah, it's, rather complicated. So I, yeah, so sorry, I got the wrong cue from your, bio. So then what is your current focus and how do we help our audience understand it with more depth?
[00:23:11] Ieva Čepaitė: Yeah, so at the moment, I'm, at Phasecraft, like I said, and, broadly they work on, I think, many different projects, and, myself, I've come in knowing adiabatic stuff, adiabatic quantum computing is linked to, in a more adiabatic So, moving away from the, I'm looking at the physical device.
[00:23:32] I'm just looking at these pulses on a more, hey, quantum gates, more abstract level, there's this algorithm called the quantum approximate optimization algorithm, which is basically in some sense, inspired by adiabatic quantum computation and there, so I'm, vaguely looking into this now and then things to do with, QAOA.
[00:23:55] Funnily, I can't say this because there is a very nice paper route that links, I don't know if this is too technical. I hope not, but
[00:24:04] Kevin Rowney: let's dive in. Let's just give it a go. So
[00:24:07] Ieva Čepaitė: that links, the error you get. from doing this QAOA, this quantum approximate algorithm. so because you're doing these short pulses, you're switching between this one pulse, different pulse, one pulse, you do it like in series.
[00:24:25] You get this error by just discretizing everything, doing these shots, basically. And this error turns out to be of the same form as a counter diabetic drive. So if you just... Oh, interesting, yeah. If you tune things a little bit, you can get for free this... It's the suppression of non diabatic errors.
[00:24:41] That's fascinating.
[00:24:43] Sebastian Hassinger: it sounds the techniques you learned from the counter diabetic, work, there's you can derive potentially, as you said, gains for free within an, an experimental setting, but you could also be feeding into, gate design or even system design.
[00:25:04] and it's, it does feel like the stage we're at right now is this, on the one hand, there's hand tuning of these very bespoke, whatever, Experimental approaches to trying to get some kind of result with a specific piece of hardware, a specific problem set. And then the other hand, we're trying to, learn from that and embed it into the development of the technology itself.
[00:25:27] So that the, systems get better, they get more capable, and eventually, it'll be easier to use these things. Hopefully.
[00:25:35] Kevin Rowney: Yeah. I, wonder, is it, it's sometimes a little bit, uncomfortable to try to speculate about the future. And I try to, with diplomacy and care, ask these sorts of questions of our guests.
[00:25:51] But I, hopefully this is not too much to ask. But could I, just get you to yourself speculate on some of the future new breakthroughs that might emerge with respect to quantum algorithms? it's a rich, deep space filled with possibility, but it's very hard for the general public to really appreciate what's going on, given it's, aggressive abstraction.
[00:26:12] Ieva Čepaitė: Yeah. it's, it is hard to say, but that's the thing. It's hard to say, because I think. My honest belief is most of the development right now we're waiting on is definitely on the hardware side. There's only so much you can do in theory and I'm vaguely optimistic because I've seen what the experiments are doing and then they're making slow but steady progress So there is there's obviously something happening and hopefully at some point we'll get to a level where You know, you can either implement some near term thing that's largely useful, in the vein of, quantum chemistry, maybe those are usually the most fair applications.
[00:26:51] Or, at some point we get to some lower enough error rates that's scaling up where you can do... You can at least envision quantum error correction, and quantum fault tolerance to be, a good, decent future. in, my, mind, I think one of the reasons why quantum machine learning took off so much, in part because there's a lot of hype surrounding AI, ML, and Nets and...
[00:27:15] I need to
[00:27:15] Sebastian Hassinger: know this.
[00:27:15] Kevin Rowney: To be sure, a compelling topic. It's not just... But I get it. Everybody's applying the AI, I don't know, spin on top of everything right now. It's a little
[00:27:25] Ieva Čepaitė: tedious. Yeah. But, but I think in part also because it's a very, clear cut way to combine, quantum and classical resources, which I think is what probably seems like a smart way to use.
[00:27:39] To use these, this near term technology, right? It's we know that we're not gonna get something exact out. Anyone who thinks that has never spoken to, someone who's building these machines, as someone who's used them at length, let's put it this way, but. It is true that even with all the noise and even with all the errors, you still get some information out.
[00:27:59] And if you work really hard, you can get a decent signal out. And so this combination of something classical and something quantum that can make use of this decent signal. that they wouldn't be able to say, get from somewhere else. I think that might, be the nearest term application.
[00:28:16] Kevin Rowney: yeah.
[00:28:17] Yeah.
[00:28:17] Sebastian Hassinger: You mentioned chemistry. You did some work, I think, in, in Singapore on chemical simulations, right? That's really cool. And do you, do you, I guess I, when I, think about quantum chemistry, computational chemistry, and quantum computing, my, I wonder, is there going to be, do we have to wait for fully, fault tolerant quantum computing, or is there an interim approach that combines some of these, variational or QAOA, maybe some analog, and, also, as you said, very clever, architectures that combine, high performance classical with, quantum resources that, that could actually be useful.
[00:28:59] Ieva Čepaitė: There's There might be avenues. One of the issues is, and I think I, maybe this is the most important thing to even state when we're talking about advantage or anything, right? Classical algorithms are good. they're really good. For most things you're trying to do, they're really, good. Even, and quantum chemistry is the one case where we need such high precision and the computations are so complex that actually, this is where, Classical machines genuinely struggle and we know they struggle just because of the precision we need for most things and so I Think it's really hard to say if you can do anything As ad hoc with quantum computers for quantum chemistry, you do need to beat the precision of classical computers.
[00:29:43] If even I've run like simulations of small molecules for myself and been like, oh, look, there's a signal I get. Clearly corresponds to the thing. this is working to some extent, but it's not giving me anything that a classical algorithm wouldn't give. in,
[00:29:59] Kevin Rowney: Yeah.
[00:29:59] Yeah. and, in, in the classical realm, there's like huge innovation happening right now in, in, classical methods for, chemistry. all the ML techniques apply to classic, Yeah. quantum, Sorry, appropriate quantum informed algorithms in the classical space.
[00:30:20] Those are advancing rapidly right now. yeah. And so it feels like there's a competition with respect to whether a quantum computer could actually exceed that. I, many speculate though that perhaps, dynamic systems would be the most killer application that the, evolution, of, subatomic systems is perhaps the era or rather the realm where the biggest advantage will be expressed.
[00:30:44] Yeah, I
[00:30:44] Ieva Čepaitė: mean, like in my mind, I tend to try to abstract away and simplify things. So in my mind, I've got it as a sort of list of, if you keep improving, compute power for, say, machine learning, then all you're really relying on is that your data is good enough. So if you've already got good enough data, then you can technically compute really complex things.
[00:31:06] if the pattern is already in there, it doesn't really matter. how long was, you can compute it well enough as long as you've got good enough data, you're fine. and with other approaches, it's it's much cleaner to say, Hey, here's the size of the matrix we can compute. Here's the approximation that we're applying here.
[00:31:24] So the limits are a bit more clear. It's tensor networks, which are the usual thing we use. like quantum systems, there people can really tell me like, Hey, if you've got this much entanglement, this big system, this whatever, I can't really do better than that, at least to this precision, right? And so quantum has to come out on top on either one of these two.
[00:31:44] So either it's the data doesn't have the right information, right? So the only way you could really predict something is using Some algorithm that's more precise, right? This is what the quantum will do, because if the data is already in there, where AI is great at doing this, we're not going to challenge that.
[00:32:03] And on the other hand, with tensor networks as well, like you need to build something that's good enough, that's large enough, that's precise enough, and then you can claim, hey, now, we've done it. Yeah, I don't know if this makes sense, but this is how I view it, which is as long as there's room to grow in one of those two spaces, then there will be a
[00:32:22] Sebastian Hassinger: competition.
[00:32:23] and it sounds you're, somewhat obliquely referring to, some work that Robert Hwang has done on, predicting quantum... properties of a system, right? I think I saw that, that was, you've looked at, or you've forked some of his code as well. we talked to, Preskill, just a couple of months ago, who's his advisor, and he brought that, work up too.
[00:32:43] So is that what you mean? if your data is correct, then you can classically using ML techniques, you can classically predict the characteristics of a, fairly complex quantum system already, because as you said, the data is right. If
[00:32:57] Ieva Čepaitė: the data is right, and if the data is good enough, in the sense of.
[00:33:01] If the data actually has the patterns you're looking for, right? So one of the reasons why this, small project that, I'm didn't realize that they knew, but, even though I posted about it, but this thing that I got inspired to do from, Robert's work, is basically The only reason I pushed it as far as I went is because I went well, if you can just do everything with AI, then just do it with AI.
[00:33:26] What's the point? Yeah. But one of the reasons why I thought it was still interesting is because, the idea is you need to have some examples from quantum systems. You need to take these measurements from quantum systems in order to then train a model in order to then get new solutions for new systems.
[00:33:44] And so assuming you need. If you need large enough, you need examples from large enough systems that you couldn't just simulate, then you still need a quantum black box or a quantum system that will give you those samples that you can then submit to a machine learning model. So there's still this interplay of you would just not have the data if you didn't have a quantum system.
[00:34:05] Computer. I'm not sure if this is true in this case, and I never pushed it far enough to check if I need like big enough, sort
[00:34:12] Sebastian Hassinger: of a big enough. That's so interesting is I, think of, quantum machine learning the way it first came into the field was this sort of, hybrid approach of making a guess, with the, QPU and then assessing that guess with the classical and going back and forth.
[00:34:31] but what you just described is suggest two different scenarios on top of that. One is using a quantum device as input into an AI model, as a, as you said, as the source of data. So you can get your, if your data is of the high quality coming out of that black box and your, AI model can actually extrapolate and get meaningful results.
[00:34:51] And then the other thing I'm wondering about is, back to the counter, the generally the, topic of kind of counter diabetic, correction, like using AI models to better control the physical quantum system that you're using for computation, right? shaping gates with things like.
[00:35:10] Counterdiabatic forces or dynamical decoupling or other sort of, I would say like extra gate kind of activity, right? The pulses around the gate that kind of helps stabilize that gate.
[00:35:23] Ieva Čepaitė: Yeah, I think people are doing this. I wouldn't be surprised, I'm sure people are doing this. I'm just haven't looked into it myself, but using machine learning to optimize pulses or, yeah, I'm
[00:35:35] Kevin Rowney: sure that this is happening.
[00:35:36] Yeah, it's yeah, no doubt.
[00:35:38] Sebastian Hassinger: Yeah, it's just a really good example of, calling back to the, your introduction of your own stuff, like the, that breadth of, spanning experimentalists, theorists, condensed matter theory, et cetera, et cetera, like, all of those realms can be leveraged in different ways to improve each other in a, way, this is, this huge co design activity that's going on right now that's really fascinating.
[00:36:01] Kevin Rowney: Yeah, this has been so interesting. I really appreciate this time, especially so in giving just a overview of this landscape, to our audience, we're just so grateful for this perspective. And, these are challenging topics to render with clarity. So yeah, props to you. Thank you so much.
[00:36:18] Thank you. Yeah, I really appreciate it. Wow. That
[00:36:21] Ieva Čepaitė: was super fun.
[00:37:06] Kevin Rowney: I really, enjoyed that. I, just thought it was really fascinating to hear the. The overview, of this contrast between these adiabatic approaches and these counter diabatic techniques. and what was especially, I think, fascinating for me is to hear of these new approaches that could harness the power of analog quantum computing systems instead of ones with gates.
[00:37:29] a lot of, potential down there. I, never really was able to, I think, see it with clarity where, the systems could, go in their full potential. So pretty
[00:37:37] Sebastian Hassinger: cool. Yeah, very cool. Absolutely. analog systems, in a sense, the, D Wave, quantum annealers are analog, because they don't have a set of gates, And they have some interesting, characteristics. It's been difficult to get, provable results out of them because, of course, they're heuristic systems, rather than, than, deterministic. there's, a lot of nuance in that. And then. In the newer systems, the, QuEra device, we just talked to Alex Keesling from QuEra a couple of episodes ago.
[00:38:11] That system currently is available as an analog Hamiltonian simulation, as a service. And obviously Dana Anderson also talked about, quantum simulations in that context as well, as the analog mode. And yeah, the, counterdiabatic approach to potentially... Providing more stability in those systems is really intriguing.
[00:38:32] and then of course, it's interesting, Yves has got such a broad set of experiences, from experimentalists to theorists to, quantum machine learning to, to these sort of, foundational techniques. It's interesting how... It sounded like there was a number of different ways that it might be applied to these analog systems, but also potentially improving the fidelity of systems or supporting a universal set of gates.
[00:38:56] So that was really interesting to hear as
[00:38:58] Kevin Rowney: well. It was. It was. I think it's really representative of the fascination I think many of us have for this space is that many of the practitioners, they have to span multiple levels of the stack, so to speak, and have a broad, background or numerous different, possibly incompatible topics of research to really grok what they're doing.
[00:39:17] So
[00:39:17] Sebastian Hassinger: cool. Yeah, I really like that aspect of all these conversations is you can really see people, straining to make connections across those disparate fields that need to be bridged in so many different ways. co design is the phrase that often comes up in those, types of scenarios.
[00:39:35] And I think that's a really prevailing theme of this phase we're in quantum computing. So fascinating stuff. Good stuff.
[00:39:44] Kevin Rowney: Okay. That's it for this episode of The New Quantum Era, a podcast by Sebastian Hassinger and Kevin Roney. Our cool theme music was composed and played by Omar Costa Hamido.
[00:39:57] Production work is done by our wonderful team over at Podfly. If you are at all like us and enjoy this rich, deep, and interesting topic, please subscribe to our podcast on whichever platform you may stream from. And even consider, if you like what you've heard today, reviewing us on iTunes. and or mentioning us on your preferred social media platforms.
[00:40:23] We're just trying to get the word out on this fascinating topic and would really appreciate your help spreading the word and building community. Thank you so much for your time.
Creators and Guests
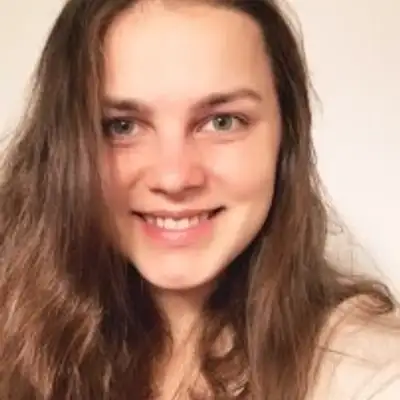
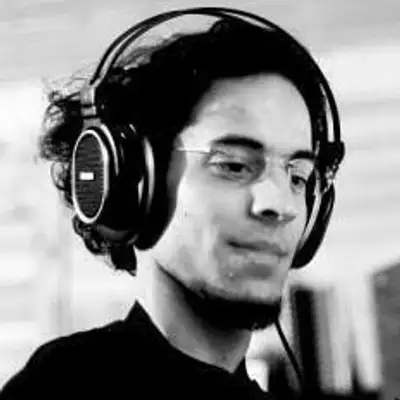
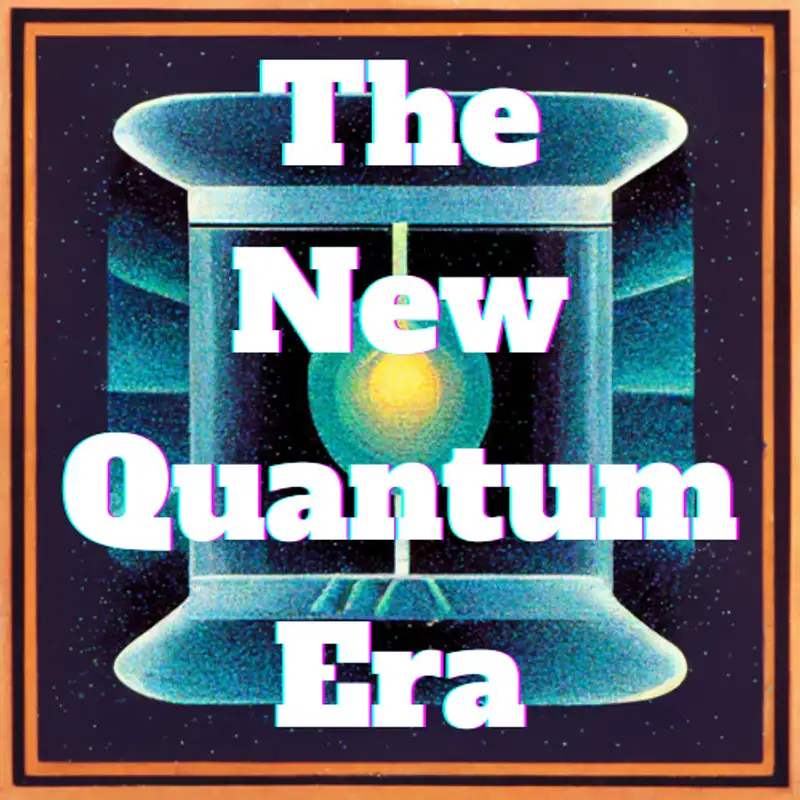